Cluster analysis: Insights into target groups, markets & products
Appinio Research · 06.12.2023 · 14min read
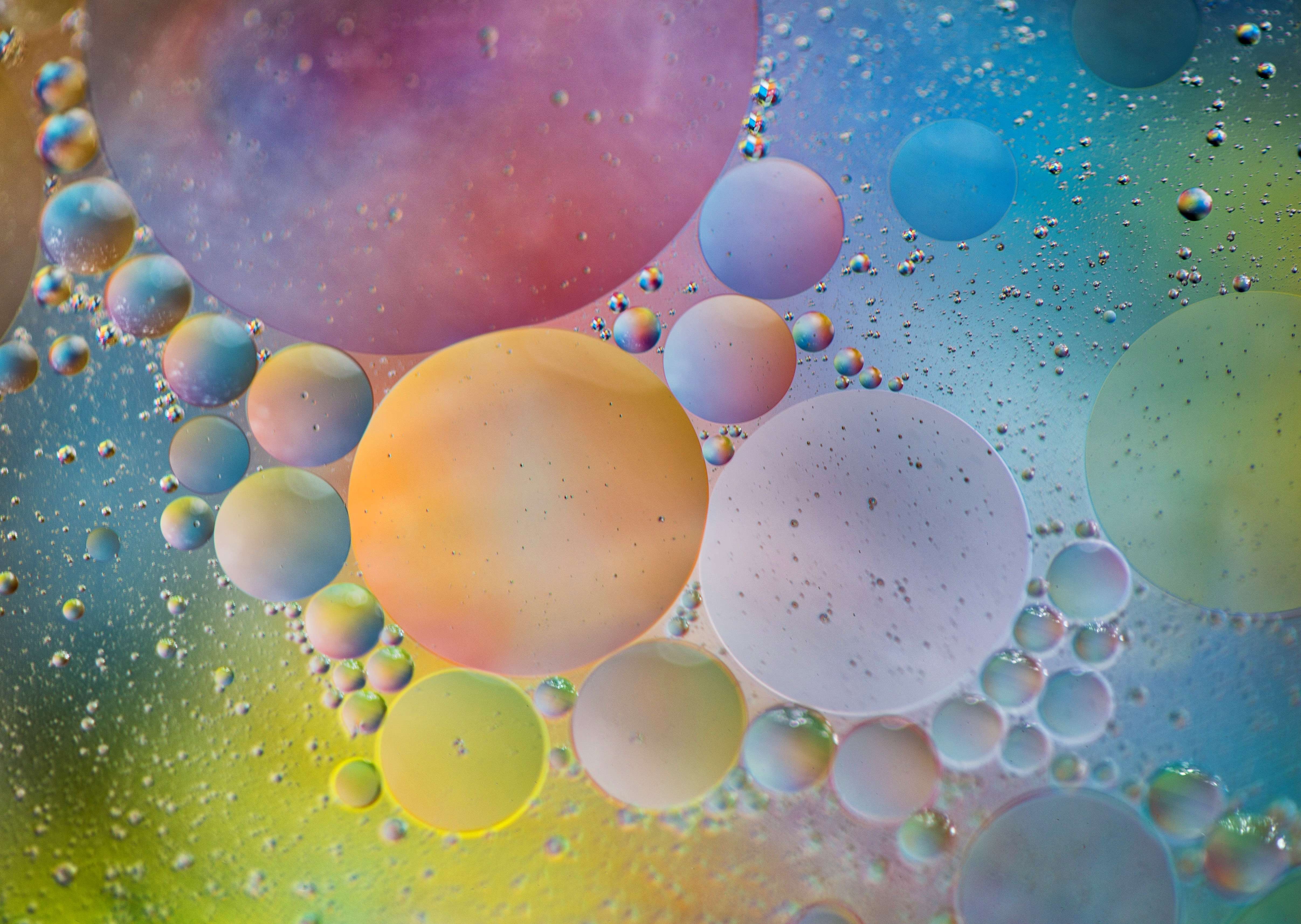
Content
Gaining profound insights into target groups and markets, fortifying customer loyalty, propelling developmental endeavors, and evaluating the risks associated with a product launch – all these feats become attainable through a great tool: cluster analysis. This method unravels patterns and correlations embedded within vast datasets.
In the following article, we delve into the essence of cluster analysis, tracing its origins, highlighting its merits for both market research and companies, delineating the prerequisites for a successful analysis, and uncovering the untapped potential that cluster analysis holds for optimizing your marketing strategies.
What is a cluster analysis?
Cluster analysis emerges as a versatile powerhouse in the realm of market research.
This statistical method enables the identification of patterns and clusters within data, where shared characteristics or properties bind elements together.
Homogeneous groups, referred to as 'clusters,' encapsulate akin data points or objects.
Through this segmentation, companies can acquire specific insights into their customers, products, or markets, subsequently translating these revelations into strategic marketing initiatives.
When does cluster analysis add value?
Cluster analysis emerges as a valuable asset for companies seeking profound market and target group insights.
Its utility becomes most pronounced when companies grapple with a substantial volume of customers or products, and categorizing them into distinct groups is a must.
This segmentation facilitates a more focused approach to diverse customer segments, fostering the development of personalized marketing strategies and target group approaches. Ultimately, cluster analysis serves as a catalyst for enhancing competitiveness, enabling companies to deploy resources more efficiently and make informed decisions in marketing and product development.
A brief history of cluster analysis in market research
The inception of cluster analysis dates back to the 1930s. However, it was during the 1950s and 1960s that diverse approaches to cluster analysis took shape, capturing the imagination of both market research and marketing spheres.
Recognizing its potential, companies embraced this analytical tool to segment customer data and pinpoint target groups, paving the way for tailored approaches and optimized marketing strategies.
The advent of computers in the 1980s marked a turning point, rendering cluster analysis more accessible and efficient.
Today, it enjoys unprecedented popularity.
Technological strides, including big data and advanced statistical software like SPSS, coupled with real-time, app-based data analysis, have elevated cluster analysis to an indispensable status for business success.
It serves as a vital element for understanding market segments, identifying customer needs, and formulating competitive advantages.
Applications of cluster analysis
Cluster analysis finds its foothold not only in market research but extends its reach to diverse fields, showcasing its versatility in classifying customers or data into homogeneous target groups.
This method proves invaluable for identifying patterns and correlations, paving the way for the development of personalized marketing strategies.
Beyond market research, cluster analysis finds application in various sectors:
- The social sciences leverage cluster analysis to segment population groups and unveil behavioral patterns.
- In healthcare, it aids in crafting personalized treatment plans for patient groups.
- The finance domain benefits from portfolio optimization and risk minimization.
- In biology, the tool is instrumental in unraveling genetic patterns and family trees, contributing to the exploration of specific causes of diseases.
- In the realm of mobility planning and logistics, it facilitates the examination of traffic flows, enabling the planning of more efficient routes.
The advantages and disadvantages of cluster analysis
Cluster analysis emerges as a transformative tool, propelling companies to new heights by offering a plethora of opportunities.
Advantages
- Recognizing patterns and structures: In the vast landscape of data, cluster analysis unveils hidden patterns and structures, providing invaluable insights.
- Target group identification and segmentation: It facilitates the precise identification and segmentation of target groups, laying the groundwork for targeted marketing strategies.
- Personalizing marketing strategies: With a focus on personalization, cluster analysis empowers companies to tailor marketing strategies, thus enhancing overall efficiency.
- Optimizing products and services: Companies can refine their products and services by leveraging the findings of cluster analysis, ensuring alignment with customer needs.
- Informed decision-making: Serving as a cornerstone for corporate strategy and marketing plans, cluster analysis provides a sound basis for informed decision-making.
Disadvantages
However, like any method, cluster analysis has its drawbacks:
- Subjectivity in cluster selection: The selection of clusters and determining their number can be subjective, introducing an element of interpretation.
- Resource-intensive for large datasets: Dealing with large datasets can be research-intensive and resource-consuming, potentially slowing down the analysis process.
- Impact of outliers: Individual data points acting as outliers may adversely affect clustering accuracy.
- Assumption risks: Analyses are susceptible to inaccuracies if built on incorrect assumptions regarding data classification.
- Risk of over-clustering: There is a risk of creating an excessive number of clusters, potentially leading to representations that are no longer truly reflective of the underlying data.
Navigating these considerations judiciously allows companies to harness the full potential of cluster analysis while being mindful of its limitations.
Clusters: a robust foundation for further analyses
The outcomes of cluster analysis serve a dual purpose.
Firstly, they provide an excellent launchpad for targeted marketing initiatives.
Secondly, these clusters form a solid groundwork for further investigations through regression analysis, factor analysis, or TURF analysis.
- Regression Analysis
This method explores relationships between individual variables, shedding light on the effectiveness or inefficacy of marketing activities. It also unravels intricate connections between distinct segments. - Factor Analysis
Aimed at simplifying complex datasets, factor analysis sifts through the intricacies to unveil the most crucial factors. This process identifies additional similarities between objects within a cluster, enhancing the depth of understanding. - TURF Analysis
Leveraging available data, TURF analysis scrutinizes the outcomes of marketing activities, delineating which product and marketing mix yields the most significant reach among customers.
By leveraging clusters as a robust database, companies can not only fine-tune their marketing strategies but also delve deeper into the intricate dynamics and relationships within their customer segments.
Essential prerequisites for effective cluster analysis
The efficacy of a cluster analysis hinges on the foundation of robust data.
For this purpose, it is imperative to normalize or scale the data, ensuring comparability and facilitating the classification into clusters.
The clusters themselves necessitate distinctly recognizable characteristics or variables. Equally pivotal is the selection of an appropriate algorithm and analysis software, such as SPSS, coupled with the critical task of determining the optimal number of clusters.
Key requirements include:
- Normalized or scaled data
A prerequisite for meaningful comparisons and cluster classification. - Clearly defined characteristics or variables
Essential for the identification and differentiation of clusters. - Appropriate algorithm
The right algorithm is key in ensuring the accuracy and relevance of the analysis. - Suitable analysis software
Leveraging advanced tools like SPSS enhances the efficiency and accuracy of the cluster analysis. - Optimal cluster number determination
Striking the right balance in determining the number of clusters is crucial for precision.
Above all, a clear comprehension of the analysis objectives is foundational. This understanding is essential for interpreting clusters meaningfully and extracting strategic insights that can steer informed decision-making.
Methodologies in Cluster Analysis
Cluster analysis employs various methodologies, or methods, depending on the objectives and data scenarios. Among the plethora of approaches, two stand out as the most common: hierarchical cluster analysis and k-means.
- Hierarchical Cluster Analysis:
- This method constructs a tree structure encompassing all data points, ranging from individual data points to larger clusters. Clusters can manifest at different hierarchical levels, with two fundamental directions: agglomerative (bottom-up) and divisive (top-down).
- Provides a comprehensive perspective, offering insights into hierarchical relationships among data points.
- k-Means Method:
- An iterative technique that categorizes data points into predefined k-clusters, determined before the analysis. The objective is to group similar data points within the same clusters, ensuring each cluster exhibits similar characteristics.
- Facilitates the identification of patterns and extraction of trends by grouping data points based on their similarities.
These methods offer distinct advantages based on the nature of the data and the analytical objectives. Selecting the most appropriate methodology is pivotal to the success and relevance of the cluster analysis.
Example of different group distributions as a result of a cluster analysis
Real-world application of cluster analysis
Consider a scenario where a company aims to connect with younger target groups, seeking a profound understanding of their needs to tailor individualized marketing initiatives.
To achieve this, the company conducts a survey gathering demographic data, including age, gender, place of residence, interests, and more.
The collected data undergoes analysis through cluster analysis to categorize customers into distinct groups.
- Data collection
Demographic information such as age, gender, location, and interests is gathered through a comprehensive survey. - Cluster analysis
Leveraging cluster analysis, the collected data is meticulously analyzed to identify patterns and commonalities. This results in the segmentation of customers into distinct groups based on shared characteristics. - Strategic insights
Armed with the segmented customer groups, the company gains valuable insights into the unique needs and preferences of younger target demographics. - Targeted marketing measures
With a nuanced understanding of each customer cluster, the company can craft targeted marketing strategies tailored to the specific characteristics and preferences of each group. - Enhanced engagement
By aligning marketing measures with the identified customer clusters, the company maximizes its outreach and engagement with the younger target groups.
In this example, cluster analysis serves as a powerful tool, enabling the company to not only comprehend the diverse needs of younger demographics but also to strategically tailor marketing initiatives, fostering a more personalized and impactful connection with their target audience.
Cluster analysis in nine steps
Cluster analysis stands out as an invaluable tool for extracting coherent patterns and groups from vast datasets, offering profound insights for refining marketing strategies and targeting specific audience segments.
Here is an overview of the typical nine-step process in cluster analysis:
- Data preparation
Before diving into the analysis, ensure the data, whether customer information or product characteristics, is meticulously collected, complete, and standardized. - Variable selection
Identify the relevant variables and characteristics for the analysis. These may include demographic data, purchasing behavior, or product features. - Data normalization
Normalize the data to enhance comparability, allowing for better scaling and extraction of different characteristics across units or value ranges. - Method selection
Choose the appropriate cluster analysis method based on the specific objectives and data characteristics. - Number of clusters determination
Decide on the optimal number of clusters to divide the data. This can be achieved through visual inspection or statistical methods like the elbow criterion. - Implementation of cluster analysis
Assign each data point to a specific cluster using statistical software such as SPSS. - Results interpretation
Analyze the formed clusters to identify distinctive features and differences between groups. Extract marketing strategies or product enhancements based on these key characteristics. - Validation and implementation of results
Critically review and validate results using internal or external validation methods. Implement targeted marketing strategies or adjust products and services to cater to the unique needs of individual clusters. - Monitoring and adaptation
Continuously monitor the effectiveness of implemented measures and adapt strategies as needed. Employ cluster analysis as an ongoing tool to identify market changes and evolving customer behaviors, ensuring flexibility in strategy adjustments.
Why companies should use cluster analysis
Cluster analysis emerges as a great tool, empowering market researchers and companies to distill intricate data into lucid and interpretable patterns.
By categorizing customers or products into clusters, this method facilitates the precise identification of target groups, paving the way for the development of tailor-made marketing strategies. This strategic approach secures you a competitive edge.
Here's why companies should leverage cluster analysis:
- Precision in target group identification
Cluster analysis allows for the precise identification of target groups, enabling companies to understand and cater to the unique needs of diverse customer segments. - Tailor-made marketing strategies
By grouping customers or products into clusters, companies can develop tailor-made marketing strategies that resonate with the specific characteristics and preferences of each cluster. - Enhanced competitive advantages
The insights derived from cluster analysis contribute to the formulation of strategies that enhance competitive advantages. This is especially crucial in navigating today's highly competitive business landscape. - Improved market understanding
Companies gain a nuanced understanding of market diversity, empowering them to navigate and respond effectively to the dynamic landscapes of various markets. - Data-driven decision-making
Harnessing cluster analysis enables companies to make data-driven decisions, ensuring strategic choices are informed and optimized for success.
In a business world marked by intense competition, the adoption of cluster analysis emerges as an invaluable asset, offering a pathway to better comprehend markets, meet customer needs, and make informed strategic decisions.
Interested in running your own study?
In our dashboard, you will find questionnaire templates that you can customize and get the insights you need to bring your brand to the next level.
Get facts and figures ðŸ§
Want to see more data insights? Our free reports are just the right thing for you!