Blending machine learning and human expertise for high-quality research data
Appinio Research · 13.02.2025 · 5min read
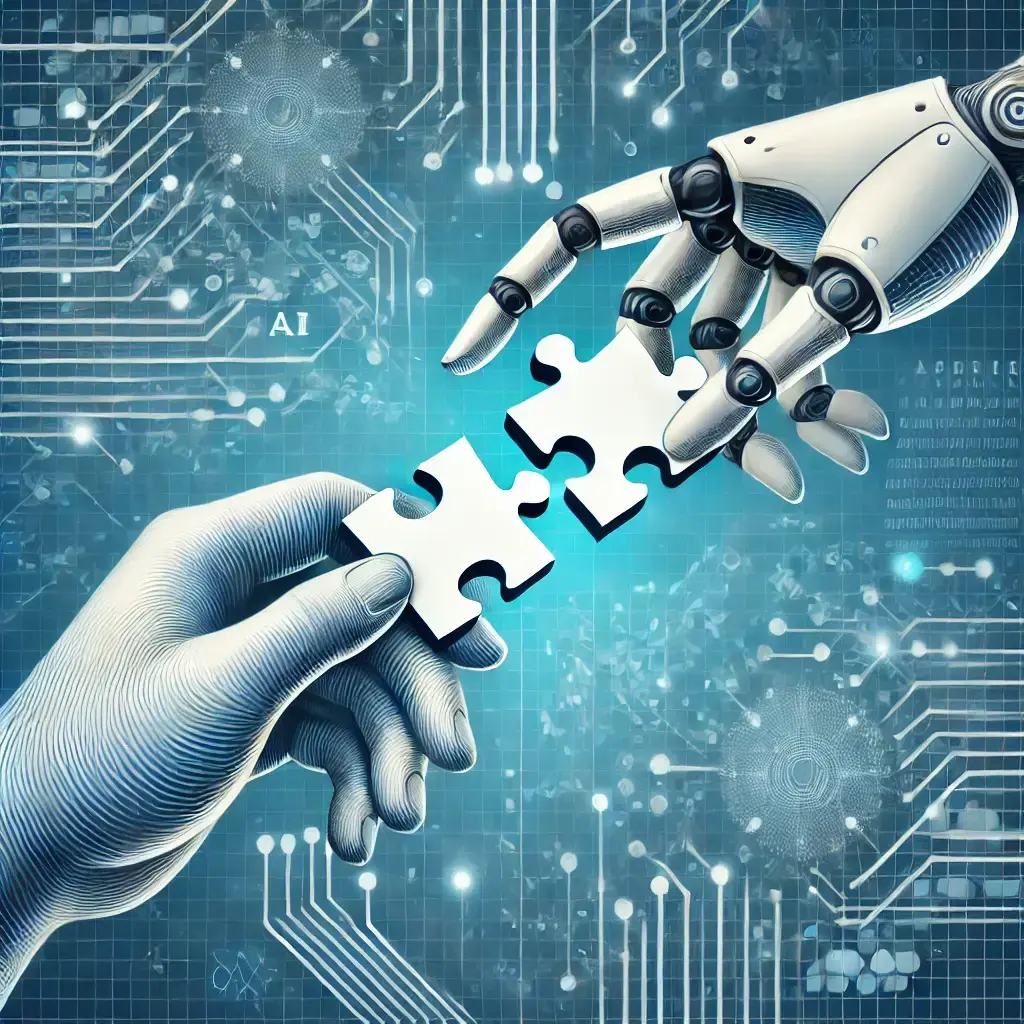
Content
At Appinio, high-quality data is the backbone of the insights we deliver. Maintaining data integrity requires a precise balance between sophisticated machine learning (ML) tools and the expertise of our research team.
How we address low-quality responses
Several factors can cause poor data: participants might become fatigued, misinterpret questions, or simply not pay attention to responses. To reduce these issues, we use an automated system that flags well-defined problems. On top of that, more advanced ML models detect more subtle patterns that may indicate low-quality submissions.
We look at common red flags, like repetitive or contradictory answers, completion times that are far too short, or inconsistencies between responses. We then apply automated checks combined with human review. This approach not only ensures that suspicious responses are carefully assessed within the context of the study, but also helps refine our ML models over time.
Striking the right balance
The real challenge is in making two things happen at the same time:
- Capturing as many low-quality responses as possible.
- Minimizing false positives (so valid data remains unscathed).
If the filters are too strict, they exclude genuine participants. Too lenient filters could let poor responses slip through. Our solution is a hybrid method: powerful ML supported by human oversight. Only when combining automated processes with expert validation can we maintain very rigorous quality standards.
Our hybrid process in action
Currently, our ML system classifies the vast majority of responses with great efficiency, labeling them as valid or invalid with a high degree of confidence. For the small number of answers that remain ambiguous, our Research Operations team steps in and reviews them manually. By doing so, we don’t only safeguard against potential errors, we also generate critical insights that help retrain and fine-tune the model.
An evolving infrastructure
We’ve built an infrastructure designed to improve continuously. User behavior changes, so our models need regular updates. We collect new data on an ongoing basis, and our system is set up to periodically retrain the model. That’s how it keeps pace with emerging patterns.
Before any retrained model goes live, we review key performance metrics and compare them to previous benchmarks. If the updated model proves more accurate without introducing bias, we can seamlessly deploy it. Otherwise, we retain the existing version. This careful approach ensures stability while still allowing for quick adaptation.
Safeguarding against bias
Throughout the process, staying fair and objective is key. When we bring ML-generated data into training, there’s always a risk of introducing or even amplifying bias. That’s where human expertise comes in—we blend algorithmic labeling with careful human review. Early input from people helps the model learn what really matters, and ongoing oversight keeps things on track, preventing unintended bias down the line.
The model
We use a strong classification model that can handle different data types and stay reliable, even with noisy data. While we’re always looking for ways to improve with more advanced methods, our current model does a great job of spotting which responses need a closer look.
When developing the model, we create new features using our research expertise, adding subtle signals that help distinguish real data from potential fraud. For evaluation, we focus on two main goals:
- High recall for low-quality responses – so we catch as many problematic answers as possible.
- High precision for valid responses – so that genuine data isn’t wrongly flagged.
Since low-quality responses are pretty rare, allowing a slightly higher false-positive rate for them can greatly reduce manual work without hurting data quality. At the same time, we set stricter thresholds for valid responses to keep legitimate data as accurate as possible.
Achieving a balanced approach
From day one, human expertise has shaped our ML-driven cleaning process, balancing quality and practicality. This collaboration between people and technology keeps our data both reliable and flexible—human oversight prevents overly strict filtering, while automation speeds up the detection of low-quality responses. With this approach, we can adapt to changing participant behaviors and consistently deliver trustworthy insights to our clients.
If you're interested in how Appinio can help your business achieve similar results, get in touch.
Get facts and figures ðŸ§
Want to see more data insights? Our free reports are just the right thing for you!