8 Types of Research Bias and How to Avoid Them?
Appinio Research · 18.10.2023 · 38min read
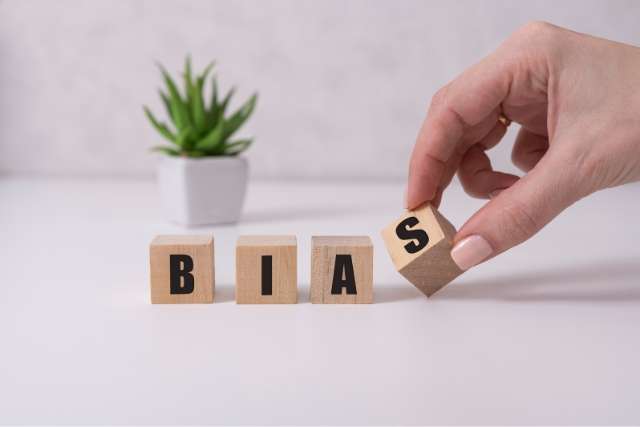
Content
Curious about how to ensure the integrity of your research? Ever wondered how research bias can impact your findings? How might it affect your data-driven decisions?
Join us on a journey through the intricate landscape of unbiased research as we delve deep into strategies and real-world examples to guide you toward more reliable insights.
What is Bias in Research?
Research bias, often simply referred to as bias, is a systematic error or deviation from the true results or inferences in research. It occurs when the design, conduct, or interpretation of a study systematically skews the findings in a particular direction, leading to inaccurate or misleading results. Bias can manifest in various forms and at different stages of the research process, and it can compromise the validity and reliability of research outcomes.
Key Aspects of Research Bias
- Systematic Error: Bias is not a random occurrence but a systematic error that consistently influences research outcomes.
- Influence on Results: Bias can lead to overestimating or underestimating effects, associations, or relationships studied.
- Unintentional or Intentional: Bias can be unintentional, stemming from flaws in study design, data collection, or analysis. In some cases, it can also be introduced intentionally, leading to deliberate distortion of results.
- Impact on Decision-Making: Research bias can have significant consequences, affecting decisions in fields ranging from healthcare and policy to marketing and academia.
Understanding and recognizing the various types and sources of bias is crucial for researchers to minimize its impact and produce credible, objective, and actionable research findings.
Importance of Avoiding Research Bias
Avoiding research bias is paramount for several compelling reasons, as it directly affects the quality and integrity of research outcomes. Here's why researchers and decision-makers should prioritize bias mitigation:
- Credibility and Trustworthiness: Research bias undermines the credibility and trustworthiness of research findings. Biased results can erode public trust, damage an organization's reputation, and hinder the acceptance of research in the scientific community.
- Informed Decision-Making: Research serves as the foundation for informed decision-making in various fields. Bias can lead to erroneous conclusions, potentially leading to misguided policies, ineffective treatments, or poor business strategies.
- Resource Allocation: Bias can result in the misallocation of valuable resources. When resources are allocated based on biased research, they may not effectively address the intended issues or challenges.
- Ethical Considerations: Introducing bias, whether intentionally or unintentionally, raises ethical concerns in research. Ethical research practices demand objectivity, transparency, and fairness in the pursuit of knowledge.
- Advancement of Knowledge: Research contributes to the advancement of knowledge and innovation. Bias hinders scientific progress by introducing errors and distorting the true nature of phenomena, hindering the development of accurate theories and solutions.
- Public Health and Safety: In fields like healthcare, bias can have life-and-death implications. Biased medical research can lead to the adoption of less effective or potentially harmful treatments, putting patient health and safety at risk.
- Economic Impact: In business and economics, biased research can result in poor investment decisions, market strategies, and financial losses. Avoiding bias is essential for achieving sound economic outcomes.
In the pursuit of unbiased research, having a robust data collection platform is crucial. Appinio offers a comprehensive solution that helps researchers gather data in a transparent and unbiased manner. With Appinio, you can access a diverse and representative pool of respondents, ensuring that your research reflects a broad spectrum of perspectives.
Appinio's platform also facilitates data collection with rigorous quality control, reducing the risk of bias at the source. By partnering with Appinio, you can enhance the integrity and credibility of your work, ultimately contributing to more reliable and impactful research outcomes.
Explore the possibilities with Appinio – book a demo today!
The importance of avoiding research bias cannot be overstated. Recognizing bias, implementing strategies to mitigate it, and promoting transparent and unbiased research practices are essential steps to ensure that research contributes meaningfully to advancing knowledge, informed decision-making, and the well-being of individuals and society as a whole.
Common Types of Research Bias
Research bias can manifest in various forms, each with unique characteristics and implications. Understanding these common types of research bias is essential for recognizing and mitigating their effects on your research.
Selection Bias
Selection bias occurs when the sample used in a study does not represent the target population, leading to distorted results. It can happen when certain groups are systematically more or less likely to be included in the study, introducing bias.
Causes of Selection Bias:
- Volunteer Bias: Participants self-select to participate in a study, and their motivations or characteristics differ from those who do not volunteer.
- Convenience Sampling: Researchers choose participants who are readily available but may not be representative of the broader population.
- Non-Response Bias: Occurs when a significant portion of selected participants does not respond or drops out during the study, potentially due to differing characteristics.
Mitigation Strategies:
- Random Sampling: Select participants randomly from the target population to ensure equal representation.
- Stratified Sampling: Divide the population into subgroups and sample proportionally from each subgroup.
- Use of Control Groups: Compare the study group to a control group to help account for potential selection bias.
Sampling Bias
Sampling bias arises when the individuals or items in your sample are not chosen randomly or are not representative of the broader population. It can lead to inaccurate generalizations and skewed conclusions.
Causes of Sampling Bias:
- Sampling Frame Issues: When the list or database used to select the sample is incomplete or outdated.
- Self-Selection: Participants choose to be part of the sample, introducing bias if their motivations differ from non-participants.
- Undercoverage: When certain groups are underrepresented in the sample due to difficulties in reaching or including them.
Mitigation Strategies:
- Random Sampling: Employ random selection methods to ensure every individual or item has an equal chance of being included.
- Stratified Sampling: Divide the population into homogeneous subgroups and sample proportionally from each subgroup.
- Quota Sampling: Set quotas for specific demographics to ensure representation.
Measurement Bias
Measurement bias occurs when the methods used to collect data are inaccurate or systematically flawed, leading to incorrect conclusions. This bias can affect both quantitative and qualitative data.
Causes of Measurement Bias:
- Instrument Flaws: When the measurement tools used are inherently unreliable or imprecise.
- Data Collection Errors: Mistakes made during data collection, such as misinterpretation of responses or inconsistent recording.
- Response Bias: Participants may provide socially desirable responses, leading to measurement errors. Next to that are various types of bias that arise from the structure of the questionnaire and psychologically influence the participants' answers. We summarized those on our Instagram:
Mitigation Strategies:
- Use Reliable Instruments: Select measurement tools that have been validated and are known for their accuracy.
- Pilot Testing: Test data collection procedures to identify and address potential sources of measurement bias.
- Blinding: Keep researchers unaware of specific measurements to minimize subjectivity.
Reporting Bias
Reporting bias involves selectively reporting results that support a particular hypothesis while ignoring or downplaying contrary findings. It can lead to a skewed representation of the evidence.
Causes of Reporting Bias:
- Publication Pressure: Researchers may prioritize publishing positive or significant results, leaving negative or inconclusive findings unreported.
- Editorial Bias: Journals may preferentially accept studies with significant results, discouraging the publication of less exciting findings.
- Confirmation Bias: Researchers may unintentionally focus on, emphasize, or interpret data that aligns with their hypotheses.
Mitigation Strategies:
- Transparent Reporting: Share all research findings, whether they support your hypotheses or not.
- Pre-Registration: Register your research design and hypotheses before data collection, reducing the temptation to selectively report.
- Peer Review: Engage in peer review to ensure a balanced and comprehensive presentation of your research.
Confirmation Bias
Confirmation bias is the tendency to seek out or interpret information in a way that confirms pre-existing beliefs or expectations. It can cloud objectivity and lead to the misinterpretation of data.
Causes of Confirmation Bias:
- Cognitive Biases: Researchers may unconsciously filter or interpret data in a way that aligns with their preconceptions.
- Selective Information Search: Researchers might seek out information that supports their hypotheses while ignoring contradictory evidence.
- Interpretation Bias: Even when presented with neutral data, researchers may interpret it to fit their expectations.
Mitigation Strategies:
- Blinding: Keep researchers unaware of the study's hypotheses to prevent bias in data interpretation.
- Objectivity Training: Train researchers to approach research with open minds and to recognize and challenge their biases.
- Diverse Perspectives: Collaborate with colleagues with different viewpoints to reduce the impact of confirmation bias.
Publication Bias
Publication bias occurs when studies with positive or significant results are more likely to be published, skewing the overall literature. Unpublished studies with negative or null findings remain hidden.
Causes of Publication Bias:
- Journal Preferences: Journals may favor publishing studies with significant results, leading to the underrepresentation of negative or null findings.
- Researcher Publication Bias: Researchers may prioritize submitting and resubmitting studies with positive results for publication.
Mitigation Strategies:
- Publication of Negative Results: Encourage publishing studies with negative or null findings.
- Meta-analysis: Combine results from multiple studies to assess the overall effect, considering both published and unpublished studies.
- Journal Policies: Support journals that promote balanced publication practices.
Recall Bias
Recall bias arises when participants in a study inaccurately remember or report past events or experiences. It can compromise the accuracy of historical data.
Causes of Recall Bias:
- Memory Decay: Memories naturally fade over time, making it challenging to recall distant events accurately.
- Social Desirability Bias: Participants may provide responses they believe are socially acceptable or favorable.
- Leading Questions: The phrasing of questions can influence participants' recollections.
Mitigation Strategies:
- Use of Objective Data Sources: Whenever possible, rely on documented records, medical charts, or other objective sources of information.
- Minimize Leading Questions: Craft questions carefully to avoid suggesting specific responses.
- Consider Timing: Be aware of how the timing of data collection may affect participants' recall.
Observer Bias
Observer bias occurs when researchers' expectations or preconceived notions influence their observations and interpretations of data. It can introduce subjectivity into the research process.
Causes of Observer Bias:
- Expectation Effects: Researchers may see what they expect or want to see in their observations.
- Interpretation Biases: Researchers may interpret ambiguous data in a way that confirms their hypotheses.
- Confirmation Bias: Researchers may selectively focus on evidence that supports their expectations.
Mitigation Strategies:
- Blinding: Keep researchers unaware of the study's hypotheses to minimize their influence on observations.
- Inter-rater Reliability: Ensure agreement among multiple observers by using consistent criteria for data collection.
- Training and Awareness: Train researchers to recognize and mitigate their biases, promoting more objective observations.
Understanding and identifying these common types of research bias is the first step toward conducting rigorous and reliable research. By implementing effective mitigation strategies and fostering a culture of transparency and objectivity, you can enhance the credibility and impact of your research. It's not just about avoiding pitfalls but also about ensuring that your findings stand up to scrutiny and contribute to the broader body of knowledge in your field.
Remember, research is a continuous journey of discovery, and the quest for unbiased, evidence-based insights is at its core. Embracing these principles will not only strengthen your research but also empower you to make more informed decisions, drive positive change, and ultimately, advance both your individual goals and the greater collective knowledge of society.
What Causes Research Bias?
Research bias can stem from various sources, and gaining a deeper understanding of these causes is vital for effectively addressing and preventing bias in your research endeavors. Let's explore these causes in detail:
Inherent Biases
Inherent biases are those that are an intrinsic part of the research process itself and can be challenging to eliminate entirely. They often result from limitations or constraints in a study's design, data collection, or analysis.
Key Characteristics:
- Inherent to Study Design: These biases are ingrained in the very design or structure of a study.
- Difficult to Eliminate: Since they are innate, completely eradicating them may not be feasible.
- Potential to Skew Findings: Inherent biases can lead to skewed or inaccurate results.
Examples of Inherent Biases:
- Sampling Bias: Due to inherent limitations in data collection methods.
- Selection Bias: As a result of constraints in participant recruitment.
- Time-Order Bias: Arising from changes over time, which may be challenging to control.
Systematic Biases
Systematic biases result from consistent errors or flaws in the research process, which can lead to predictable patterns of deviation from the truth. Unlike inherent biases, systematic biases can be addressed with deliberate efforts.
Key Characteristics:
- Consistent Patterns: These biases produce recurring errors or distortions.
- Identifiable Sources: The sources of systematic biases can often be pinpointed and addressed.
- Amenable to Mitigation: Conscious adjustments can reduce or eliminate systematic biases.
Examples of Systematic Biases:
- Measurement Bias: When measurement tools are systematically flawed, leading to inaccuracies.
- Reporting Bias: Stemming from the selective reporting of results to favor certain outcomes.
- Confirmation Bias: Arising from researchers' preconceived notions or hypotheses.
Non-Systematic Biases
Non-systematic biases are random errors that can occur in the research process but are neither consistent nor predictable. They introduce variability and can affect individual data points but may not systematically impact the overall study.
Key Characteristics:
- Random Occurrence: Non-systematic biases are not tied to specific patterns or sources.
- Unpredictable: They may affect one data point but not another unexpectedly.
- Potential for Random Variation: Non-systematic biases introduce noise into data.
Examples of Non-Systematic Biases:
- Sampling Error: Natural fluctuations in data points due to random chance.
- Non-Response Bias: When non-responders differ from responders randomly.
Cognitive Biases
Cognitive biases are biases rooted in human psychology and decision-making processes. They can influence how researchers perceive, interpret, and make sense of data, often unconsciously.
Key Characteristics:
- Psychological Origin: Cognitive biases originate from the way our brains process information.
- Subjective Interpretation: They affect how researchers subjectively interpret data.
- Affect Decision-Making: Cognitive biases can influence researchers' decisions throughout the research process.
Examples of Cognitive Biases:
- Confirmation Bias: Seeking information that confirms pre-existing beliefs.
- Anchoring Bias: Relying too heavily on the first piece of information encountered.
- Hindsight Bias: Seeing events as having been predictable after they've occurred.
Understanding these causes of research bias is crucial for researchers at all stages of their work. It enables you to identify potential sources of bias, take proactive measures to minimize bias and foster a research environment that prioritizes objectivity and rigor. By acknowledging the inherent biases in research, recognizing systematic and non-systematic biases, and being aware of the cognitive biases that can affect decision-making, you can conduct more reliable and credible research.
How to Detect Research Bias?
Detecting research bias is a crucial step in maintaining the integrity of your study and ensuring the reliability of your findings. Let's explore some effective methods and techniques for identifying bias in your research.
Data Analysis Techniques
Utilizing appropriate data analysis techniques is crucial in detecting and addressing research bias. Here are some strategies to consider:
- Statistical Analysis: Employ rigorous statistical methods to examine the data. Look for anomalies, inconsistencies, or patterns that may indicate bias, such as skewed distributions or unexpected correlations.
- Sensitivity Analysis: Conduct sensitivity analyses by varying key parameters or assumptions in your analysis. This helps assess the robustness of your results and identifies whether bias may be influencing your findings.
- Subgroup Analysis: If your study involves diverse groups or populations, perform subgroup analyses to explore whether bias may be affecting specific subsets differently.
Peer Review
Peer review is a fundamental process for evaluating research and identifying potential bias. Here's how it can assist in detecting bias:
- External Evaluation: Involve independent experts in your field who can objectively assess your research methods, data, and interpretations. They may identify overlooked sources of bias or offer suggestions for improvement.
- Bias Assessment: Ask peer reviewers specifically to scrutinize your study for any signs of bias. Encourage them to assess the transparency of your methods and reporting.
- Replicability: Peer reviewers can also assess the replicability of your study, ensuring that others can reproduce your findings independently.
Cross-Validation
Cross-validation is a technique that involves comparing the results of your research with external or independent sources to identify potential bias:
- External Data Sources: Compare your findings with data from external sources, such as government statistics, industry reports, or previous research. Significant disparities may signal bias.
- Expert Consultation: Seek feedback from experts who are not directly involved in your research. Their impartial perspectives can help identify any biases in your study design, data collection, or interpretation.
- Historical Comparisons: If applicable, compare your current research with historical data to assess whether trends or patterns have changed over time, which could indicate bias.
By employing these methods and techniques, you can proactively detect and address research bias, ultimately enhancing the credibility and reliability of your research findings.
How to Avoid Research Bias?
Effectively avoiding research bias is a fundamental aspect of conducting high-quality research. Implementing specific strategies can help researchers minimize the impact of bias and enhance the validity and reliability of their findings. Let's delve into these strategies in detail:
1. Randomization
Randomization is a method used to allocate participants or data points to different groups or conditions in an entirely random way. It helps ensure that each participant has an equal chance of being assigned to any group, reducing the potential for bias in group assignments.
Key Aspects:
- Random Assignment: Randomly assigning participants to experimental or control groups.
- Equal Opportunity: Ensuring every participant has an equal likelihood of being in any group.
- Minimizing Bias: Reduces the risk of selection bias by distributing potential biases equally across groups.
Benefits:
- Balanced Groups: Randomization creates comparable groups in terms of potential confounding variables.
- Minimizes Selection Bias: Eliminates researcher or participant biases in group allocation.
- Enhanced Causality: Strengthens the ability to make causal inferences from research findings.
Methods:
- Simple Randomization: Assign participants or data points to groups using a random number generator or drawing lots.
- Stratified Randomization: Divide the population into subgroups based on relevant characteristics (e.g., age, gender) and then randomly assign within those subgroups.
- Blocked Randomization: Create blocks of participants, ensuring each block contains an equal number from each group.
Example:
In a clinical trial testing a new drug, researchers use simple randomization to allocate participants into two groups: one receiving the new drug and the other receiving a placebo. This helps ensure that patient characteristics, such as age or gender, do not systematically favor one group over another, minimizing bias in the study's results.
2. Blinding and Double-Blinding
Blinding involves keeping either the participants or the researchers (single-blinding) or both (double-blinding) unaware of certain aspects of the study, such as group assignments or treatment conditions. This prevents the introduction of bias due to expectations or knowledge of the study's hypotheses.
Key Aspects:
- Single-Blinding: Either participants or researchers are unaware of crucial information.
- Double-Blinding: Both participants and researchers are unaware of crucial information.
- Placebo Control: Often used in pharmaceutical research to ensure blinding.
Benefits:
- Minimizes Observer Bias: Researchers' expectations do not influence data collection or interpretation.
- Prevents Participant Bias: Participants' awareness of their group assignment does not affect their behavior or responses.
- Enhances Study Validity: Blinding reduces the risk of bias influencing study outcomes.
Methods:
- Use of Placebos: In clinical trials, a placebo group is often included to maintain blinding.
- Blinding Procedures: Establish protocols to ensure that those who need to be blinded are kept unaware of relevant information.
- Blinding Verification: Conduct assessments to confirm that blinding has been maintained throughout the study.
Example:
In a double-blind drug trial, neither the participants nor the researchers know whether they are receiving or administering the experimental drug or a placebo. This prevents biases in reporting and evaluating the drug's effects, ensuring that results are objective and reliable.
3. Standardization of Procedures
Standardization involves creating and following consistent, well-defined procedures throughout a study. This ensures that data collection, measurements, and interventions are carried out uniformly, minimizing potential sources of bias.
Key Aspects:
- Detailed Protocols: Developing clear and precise protocols for data collection or interventions.
- Consistency: Ensuring that all research personnel adhere to the established procedures.
- Reducing Variability: Minimizing variation in how processes are carried out.
Benefits:
- Increased Reliability: Standardized procedures lead to more consistent and reliable data.
- Minimized Measurement Bias: Reduces the likelihood of measurement errors or inconsistencies.
- Easier Replication: Standardization facilitates replication by providing a clear roadmap for future studies.
Methods:
- Protocol Development: Create detailed step-by-step protocols for data collection, interventions, or experiments.
- Training: Train all research personnel thoroughly on standardized procedures.
- Quality Control: Implement quality control measures to monitor and ensure adherence to protocols.
Example:
In a psychological study, researchers standardize the procedure for administering a cognitive test to all participants. They use the same test materials, instructions, and environmental conditions for every participant to ensure that the data collected are not influenced by variations in how the test is administered.
4. Sample Size Considerations
Sample size considerations involve determining the appropriate number of participants or data points needed for a study. Inadequate sample sizes can lead to underpowered studies that fail to detect meaningful effects, while excessively large samples can be resource-intensive without adding substantial value.
Key Aspects:
- Power Analysis: Calculating the required sample size based on expected effect sizes and desired statistical power.
- Effect Size Considerations: Ensuring the sample size is sufficient to detect the effect size of interest.
- Resource Constraints: Balancing the need for a larger sample with available resources.
Benefits:
- Improved Statistical Validity: Adequate sample sizes increase the likelihood of detecting actual effects.
- Generalizability: Larger samples enhance the generalizability of study findings to the target population.
- Resource Efficiency: Avoiding extensive samples conserves resources.
Methods:
- Power Analysis Software: Use statistical software to perform power analyses.
- Pilot Studies: Conduct pilot studies to estimate effect sizes and inform sample size calculations.
- Consider Practical Constraints: Factor in time, budget, and other practical limitations when determining sample sizes.
Example:
In a medical research study evaluating the efficacy of a new treatment, researchers conduct a power analysis to determine the required sample size. This analysis considers the expected effect size, desired level of statistical power, and available resources to ensure that the study can reliably detect the treatment's effects.
5. Replication
Replication involves conducting the same study or experiment multiple times to assess the consistency and reliability of the findings. Replication is a critical step in research, as it helps validate the results and ensures that they are not due to chance or bias.
Key Aspects:
- Exact or Conceptual Replication: Replicating the study with the same methods (exact) or similar methods addressing the same research question (conceptual).
- Independent Replication: Replication by different research teams or in other settings.
- Enhanced Confidence: Replication builds confidence in the robustness of research findings.
Benefits:
- Enhanced Reliability: Replicated findings are more reliable and less likely to be influenced by bias.
- Verification of Results: Replication verifies the validity of initial study results.
- Error Detection: Identifies potential sources of bias or errors in the original study.
Methods:
- Plan for Replication: Include replication as part of the research design from the outset.
- Collaboration: Collaborate with other researchers or research teams to conduct independent replications.
- Transparent Reporting: Clearly document replication methods and results for transparency.
Example:
A psychology study that originally reported a significant effect of a particular intervention on memory performance is replicated by another research team using the same methods and procedures. If the replication study also finds a significant impact, it provides additional support for the initial findings and reduces the likelihood of bias influencing the results.
6. Transparent Reporting
Transparent reporting involves thoroughly documenting all aspects of a research study, from its design and methodology to its results and conclusions. Transparent reporting ensures that other researchers can assess the study's validity and detect any potential sources of bias.
Key Aspects:
- Comprehensive Documentation: Detailed reporting of study design, procedures, data collection, and analysis.
- Open Access to Data: Sharing data and materials to allow for independent verification and analysis.
- Disclosure of Conflicts: Transparent reporting includes disclosing any potential conflicts of interest that could introduce bias.
Benefits:
- Accountability: Transparent reporting holds researchers accountable for their methods and results.
- Enhanced Credibility: Transparent research is more credible and less likely to be influenced by bias.
- Reproducibility: Other researchers can replicate and verify study findings.
Methods:
- Use of Reporting Guidelines: Follow established reporting guidelines specific to your field (e.g., CONSORT for clinical trials, STROBE for observational studies).
- Data Sharing: Make research data and materials available to others through data repositories or supplementary materials.
- Peer Review: Engage in peer review to ensure clear and comprehensive reporting.
Example:
A scientific journal article reporting the results of a research study includes detailed descriptions of the study design, methods, statistical analyses, and potential limitations. The authors also provide access to the raw data and materials used in the study, allowing other researchers to assess the study's validity and potential bias. This transparent reporting enhances the credibility of the research.
Real-World Examples of Research Bias
To better understand the pervasive nature of research bias and its implications, let's delve into additional real-world examples that illustrate various types of research bias beyond those previously discussed.
Pharmaceutical Industry Influence on Clinical Trials
Bias Type: Funding Bias, Sponsorship Bias
Example: The pharmaceutical industry often sponsors clinical trials to evaluate the safety and efficacy of new drugs. In some cases, studies sponsored by pharmaceutical companies have been found to report more favorable outcomes for their products compared to independently funded research.
Explanation: Funding bias occurs when the financial interests of the sponsor influence study design, data collection, and reporting. In these instances, there may be pressure to emphasize positive results or downplay adverse effects to promote the marketability of the drug.
Impact: This bias can have severe consequences for patient safety and public health, as it can lead to the approval and widespread use of drugs that may not be as effective or safe as initially reported.
Social Desirability Bias in Self-reported Surveys
Bias Type: Response Bias
Example: Researchers conducting surveys on sensitive topics such as drug use, sexual behavior, or income levels often encounter social desirability bias. Respondents may provide answers they believe are socially acceptable or desirable rather than accurate.
Explanation: Social desirability bias is rooted in the tendency to present oneself in a favorable light. Respondents may underreport stigmatized behaviors or overreport socially acceptable ones, leading to inaccurate data.
Impact: This bias can compromise the validity of survey research, especially in areas where honest reporting is crucial for public health interventions or policy decisions.
Non-Publication of Negative Clinical Trials
Bias Type: Publication Bias
Example: Clinical trials with negative or null results are less likely to be published than those with positive findings. This leads to an overrepresentation of studies showing treatment efficacy and an underrepresentation of trials indicating no effect.
Explanation: Publication bias occurs because journals often preferentially accept studies with significant results, while researchers and sponsors may be less motivated to publish negative findings. This skews the evidence base and can result in the overuse of specific treatments or interventions.
Impact: Patients and healthcare providers may make decisions based on incomplete or biased information, potentially exposing patients to ineffective or even harmful treatments.
Gender Bias in Medical Research
Bias Type: Gender Bias
Example: Historically, medical research has been biased toward male subjects, leading to a limited understanding of how diseases and treatments affect women. Clinical trials and studies often fail to include a representative number of female participants.
Explanation: Gender bias in research arises from the misconception that results from male subjects can be generalized to females. This bias can lead to treatments and medications that are less effective or safe for women.
Impact: Addressing gender bias is crucial for developing healthcare solutions that account for the distinct biological and physiological differences between genders and ensuring equitable access to effective treatments.
Political Bias in Climate Change Research
Bias Type: Confirmation Bias, Political Bias
Example: In climate change research, political bias can influence the framing, interpretation, and reporting of findings. Researchers aligned with certain political ideologies may downplay or exaggerate the significance of climate change based on their preconceptions.
Explanation: Confirmation bias comes into play when researchers seek data or interpretations that align with their political beliefs. This can result in less objective research and more susceptible to accusations of bias.
Impact: Political bias can undermine public trust in scientific research, impede policy-making, and hinder efforts to address critical issues such as climate change.
These diverse examples of research bias highlight the need for robust safeguards, transparency, and peer review in the research process. Recognizing and addressing bias is essential for maintaining the integrity of scientific inquiry and ensuring that research findings can be trusted and applied effectively.
Conclusion for Research Bias
Understanding and addressing research bias is critical in conducting reliable and trustworthy research. By recognizing the various types of bias, whether they are inherent, systematic, non-systematic, or cognitive, you can take proactive measures to minimize their impact. Strategies like randomization, blinding, standardization, and transparent reporting offer powerful tools to enhance the validity of your research.
Moreover, real-world examples highlight the tangible consequences of research bias and emphasize the importance of conducting research with integrity. Whether you're in the world of science, healthcare, marketing, or any other field, the pursuit of unbiased research is essential for making informed decisions that drive success. So, keep these insights in mind as you embark on your next research journey, and remember that a commitment to objectivity will always lead to better, more reliable outcomes.
How to Prevent Bias in Research?
Are you tired of lengthy, expensive, and potentially biased research processes? Appinio, the real-time market research platform, is here to revolutionize how you gather consumer insights. Say goodbye to research bias and hello to rapid, data-driven decision-making.
Here's why you should choose Appinio:
- Fast and Precise: Get from questions to insights in minutes, ensuring you have the most up-to-date information for your decisions.
- User-Friendly: No need for a PhD in research – our platform is so intuitive that anyone can use it to gather valuable insights.
- Global Reach: Survey your target audience from over 90 countries and define precise target groups from a pool of 1200+ characteristics.
Join the loop 💌
Be the first to hear about new updates, product news, and data insights. We'll send it all straight to your inbox.