Multistage Sampling: Definition, Guide, Examples
Appinio Research · 01.08.2024 · 26min read
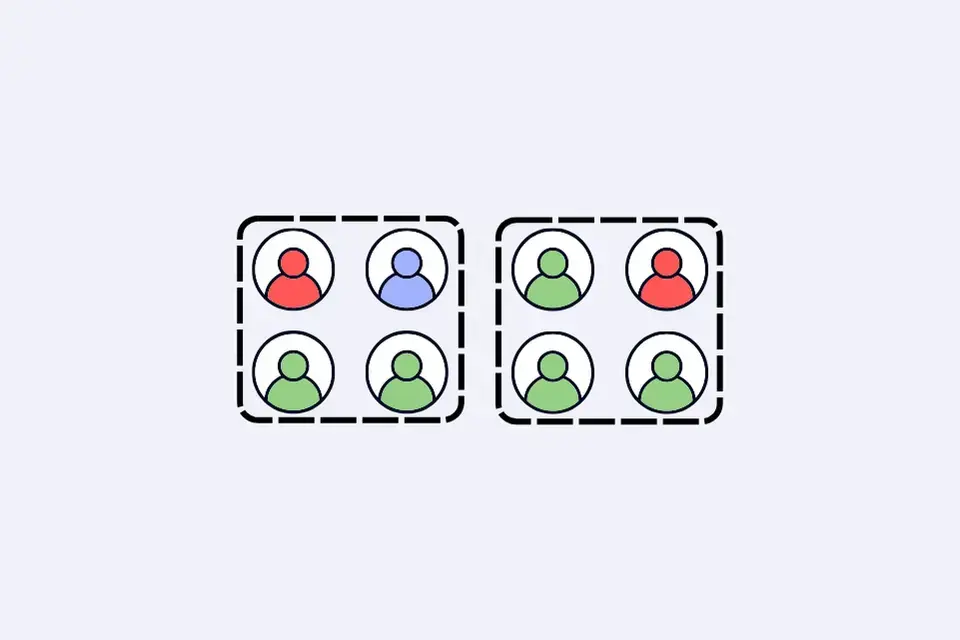
Content
Have you ever wondered how researchers manage to study large, diverse populations without surveying every single person? Multistage sampling is the answer. This guide will take you through the ins and outs of multistage sampling, a versatile and efficient technique researchers use to gather accurate data from vast populations. By breaking down the process into manageable stages, multistage sampling ensures that studies are both practical and cost-effective.
Whether you're new to the concept or looking to deepen your understanding, this guide will provide you with everything you need to know about designing, implementing, and analyzing multistage samples. From the basic principles to advanced methodologies, you'll learn how to apply this powerful tool in your own research, ensuring that your samples are representative and your findings are reliable.
What is Multistage Sampling?
Multistage sampling is a sophisticated and flexible sampling technique that involves selecting samples in multiple stages. Each stage progressively narrows down the sample from a large, broad population to smaller, more specific units. This method is particularly useful for studies involving large and geographically dispersed populations, where conducting a simple random sample would be impractical and costly.
How Multistage Sampling Works
Multistage sampling involves selecting samples in multiple stages, starting with larger groups and progressively moving to smaller units. This hierarchical approach can be broken down as follows:
- Primary Stage: Large units or clusters are selected from the population.
- Secondary Stage: Smaller units are selected within the chosen clusters.
- Further Stages: Additional stages may be added, selecting even smaller units until reaching the final units for data collection.
Characteristics of Multistage Sampling
- Hierarchical Structure: The sampling process is structured in layers, each narrowing down the population step by step.
- Flexibility: Different sampling methods can be applied at each stage, allowing for tailored approaches based on the specific needs of each level.
- Efficiency: By focusing on progressively smaller units, multistage sampling reduces logistical challenges and costs associated with sampling large populations.
Importance of Multistage Sampling in Research
Multistage sampling plays a critical role in research, particularly in studies involving large and diverse populations.
- Cost-Effectiveness: Multistage sampling significantly reduces the costs associated with data collection by narrowing down the sample size in stages rather than attempting to sample the entire population at once.
- Practicality: It is often impractical to conduct a simple random sample on a very large or geographically dispersed population. Multistage sampling makes it feasible to gather representative data in such scenarios.
- Improved Accuracy: By breaking down the sampling process into stages, researchers can control for variability within each stage, leading to more accurate and reliable results.
- Flexibility in Design: Researchers can adapt the sampling methods used at each stage to fit the specific characteristics and requirements of their study, ensuring a more tailored and effective sampling approach.
- Manageability: Handling smaller subsets of the population at each stage simplifies the data collection process, making it more manageable and less prone to errors.
- Scalability: Multistage sampling is scalable, making it suitable for both small and large studies. Researchers can easily adjust the number of stages and the size of sampling units based on the scope of their research.
- Comprehensive Data Collection: This method allows for detailed data collection at each stage, ensuring that all relevant subgroups within the population are adequately represented.
- Reduced Sampling Bias: By using a combination of different sampling techniques at various stages, multistage sampling minimizes the risk of bias that can occur with single-stage sampling methods.
- Applicability Across Disciplines: Multistage sampling is versatile and can be applied across various research fields, including public health, education, social sciences, and market research.
Multistage Sampling Comparison with Other Methods
- Simple Random Sampling vs. Multistage Sampling: While simple random sampling provides a straightforward approach, it can be impractical for large populations. Multistage sampling offers a more feasible solution by breaking down the process into stages.
- Systematic Sampling vs. Multistage Sampling: Systematic sampling is easy to implement but may miss important variations within the population. Multistage sampling, with its hierarchical approach, can capture more detailed information.
- Stratified Sampling vs. Multistage Sampling: Stratified sampling ensures the representation of specific subgroups but can be complex to organize. Multistage sampling offers a balance by allowing for different sampling methods at each stage.
- Cluster Sampling vs. Multistage Sampling: Cluster sampling simplifies data collection by focusing on entire clusters, but it may not be as accurate if clusters are not representative. Multistage sampling refines this approach by adding additional stages to improve accuracy.
Potential Drawbacks
- Complexity in Implementation: The multiple stages involved can make the design and execution of the sampling plan more complicated, requiring careful planning and coordination.
- Increased Risk of Sampling Error: Each additional stage introduces the potential for error, which can accumulate and affect the overall accuracy of the results if not managed properly.
- Need for Larger Sample Sizes: Multistage sampling often requires larger sample sizes to achieve the same level of precision as simpler methods, which can counteract some of its cost benefits.
- Resource Intensive: Despite overall cost efficiency, the detailed planning and multiple stages can be resource-intensive in terms of time, manpower, and expertise required.
- Potential Bias: If the selection process at any stage is not truly random or representative, it can introduce bias that affects the validity of the findings.
- Difficulty in Analysis: Analyzing data from multistage samples can be more complex due to the hierarchical nature of the data, requiring advanced statistical techniques and software.
- Loss of Detail: At each stage of sampling, there is a risk of losing finer details about the population, which can affect the granularity of the findings.
- Dependency on Accurate Sampling Frames: The effectiveness of multistage sampling depends heavily on the availability and accuracy of sampling frames at each stage, which may not always be reliable or complete.
By weighing the advantages and disadvantages, you can make an informed decision about whether multistage sampling suits your research project. This understanding will also help you to anticipate and address potential challenges, ensuring the success of your study.
Stages of Multistage Sampling
The multistage sampling process involves several stages, each designed to progressively narrow down the sample to a manageable and representative size. By breaking the sampling process into multiple stages, researchers can effectively handle large and diverse populations. Let's delve into each stage in detail to understand how it contributes to the overall methodology.
Primary Stage: Selection of Primary Sampling Units (PSUs)
The primary stage of multistage sampling is crucial as it sets the foundation for the entire sampling process. In this stage, large units, known as primary sampling units (PSUs), are selected from the overall population. These units can be geographical areas, institutions, or other large entities relevant to the study.
- Representativeness: PSUs should be chosen to ensure they accurately represent the broader population. This can involve using random or stratified sampling methods to select PSUs.
- Practicality: The size and scope of PSUs should be practical for the study's resources and objectives. For instance, selecting too large an area might make subsequent stages unmanageable.
- Homogeneity and Heterogeneity: Depending on the study's goals, PSUs can be chosen for their homogeneity (similarity within each PSU) or heterogeneity (diversity within each PSU) to capture different aspects of the population.
Example: In a national health survey, PSUs might be large geographical regions like states or provinces. These regions are selected to provide a broad overview of health conditions across different parts of the country.
Secondary Stage: Selection of Secondary Sampling Units (SSUs)
Once PSUs are selected, the process moves to the secondary stage, where smaller units within the PSUs are chosen. These secondary sampling units (SSUs) help further narrow down the sample.
- Nested Sampling: SSUs should be nested within the selected PSUs. For example, if PSUs are states, SSUs could be counties or districts within those states.
- Sampling Methods: Various sampling methods, such as random or systematic sampling, can be used to select SSUs. The choice depends on the study's design and objectives.
- Population Density: In densely populated PSUs, SSUs might be smaller in size to manage the volume of data, while in sparsely populated PSUs, larger SSUs might be chosen.
Example: In the context of an educational study, if PSUs are school districts, SSUs could be individual schools within those districts. The selection of schools would aim to capture the diversity and characteristics of the educational environment within each district.
Tertiary and Subsequent Stages (if applicable)
In some studies, additional stages are necessary to further refine the sample. These tertiary and subsequent stages follow the same principles as the primary and secondary stages but focus on even smaller units within the previously selected SSUs.
- Further Nesting: Each additional stage involves selecting smaller units within previously chosen SSUs. This continues until the sample is refined to the desired level of granularity.
- Resource Allocation: With each stage, resources must be carefully managed to ensure the sampling process remains feasible and practical.
- Consistency in Methodology: To avoid introducing bias, it's crucial to maintain consistency in the sampling methods used at each stage.
Example: Continuing with the educational study, if SSUs are individual schools, tertiary units could be classes within those schools. Further stages might involve selecting students within those classes, ensuring a comprehensive and detailed sample.
Final Stage: Selection of the Ultimate Sampling Units (USUs)
The final stage of multistage sampling involves selecting the ultimate sampling units (USUs), which are the smallest units from which data will be directly collected. This stage is critical as it determines the actual participants or elements that will be included in the study.
- Detailed Data Collection: At this stage, the focus is gathering detailed data from the selected units. The accuracy and reliability of this data are paramount.
- Minimizing Bias: Ensuring that the selection of USUs is random and unbiased is crucial to the validity of the study's findings.
- Logistical Feasibility: The final units should be manageable in terms of data collection logistics, considering factors such as location, accessibility, and respondent availability.
Example: In a public health survey, if the tertiary units are individual households within selected neighborhoods, the USUs would be individual members of those households. Researchers would then collect detailed health information from these individuals.
By understanding and effectively implementing each stage of multistage sampling, you can ensure that your samples are representative, manageable, and capable of providing accurate and reliable data for your studies.
Multistage Sampling Methods
Choosing the proper method for each stage of multistage sampling is crucial to ensure the sample's representativeness and accuracy. Various sampling techniques can be employed at different stages, each with strengths and considerations.
Simple Random Sampling
Simple random sampling is one of the most straightforward and widely used methods. It involves selecting units from a population in such a way that every unit has an equal chance of being chosen. This method is often employed at various stages of multistage sampling to ensure randomness and reduce bias.
How It Works:
- At each stage, a random selection of units is made from the larger group.
- Random number generators or lottery methods can be used to ensure that each unit has an equal probability of selection.
Advantages:
- Simplicity: Easy to understand and implement.
- Unbiased: Reduces selection bias by giving each unit an equal chance of being included.
- Representative: When adequately executed, it can yield a representative sample.
Considerations:
- Population Size: Requires a complete list of the population, which can be challenging for large groups.
- Feasibility: May not be practical for very large or dispersed populations without adequate resources.
Systematic Sampling
Systematic sampling involves selecting units at regular intervals from an ordered list. This method is often used in multistage sampling to simplify the selection process while still maintaining a degree of randomness.
How It Works:
- Start by randomly selecting a starting point within the first k units of the population list.
- After that, select every kth unit from the list, where k is the interval determined by dividing the population size by the desired sample size.
Advantages:
- Efficiency: Easier and faster to implement compared to simple random sampling.
- Practicality: Ideal for large populations where a complete list is available.
- Even Coverage: Ensures an even distribution of samples across the population.
Considerations:
- List Order: The order of the list should not have a systematic pattern that could bias the sample.
- Interval Size: Choosing the right interval size (k) is crucial for achieving a representative sample.
Stratified Sampling
Stratified sampling involves dividing the population into distinct subgroups, or strata, and then randomly sampling from each stratum. This method is handy when the population is heterogeneous and different subgroups need to be represented proportionally.
How It Works:
- Identify relevant strata based on key characteristics (e.g., age, gender, socio-economic status).
- Perform random sampling within each stratum at each stage.
Advantages:
- Increased Precision: Ensures all subgroups are adequately represented, improving the accuracy of results.
- Reduced Variability: Can minimize sampling error by controlling for specific variables.
- Targeted Sampling: Allows for targeted analysis of specific subgroups.
Considerations:
- Strata Definition: Defining appropriate strata is critical and requires detailed knowledge of the population.
- Complexity: More complex to design and implement compared to simple random sampling.
Cluster Sampling
Cluster sampling involves dividing the population into clusters, randomly selecting some clusters, and then sampling all or randomly selecting units within those clusters. This method is particularly efficient for geographically dispersed populations.
How It Works:
- Divide the population into clusters (e.g., neighborhoods, schools, villages).
- Randomly select a certain number of clusters.
- Sample units within selected clusters.
Advantages:
- Cost-Effective: Reduces travel and administrative costs by concentrating data collection within selected clusters.
- Practical: Suitable for large and geographically dispersed populations.
- Simplified Logistics: Easier to manage data collection within defined clusters.
Considerations:
- Cluster Homogeneity: Within-cluster homogeneity can increase sampling error if clusters are not diverse.
- Number of Clusters: Choosing too few clusters can lead to unrepresentative samples.
By understanding and applying these different sampling methods at each stage of multistage sampling, you can tailor your approach to best fit your study's specific needs and constraints, ensuring a more accurate and reliable sample.
How to Implement Multistage Sampling?
Implementing multistage sampling involves a systematic approach to ensure that each stage of the sampling process is executed effectively and efficiently. This requires careful planning, adherence to protocols, and the use of appropriate tools and software to manage and analyze the data.
How to Conduct Multistage Sampling?
Multistage sampling involves a series of steps designed to ensure the sampling process is thorough and yields accurate results.
- Define the Population and Objectives: Clearly articulate the population you are studying and your research objectives. This sets the foundation for your sampling plan.
- Develop a Detailed Sampling Plan: Outline the stages of your sampling process, including the methods used at each stage and the criteria for selecting sampling units.
- Prepare a Sampling Frame: Compile a comprehensive list of all potential sampling units within your defined population. Ensure that this list is accurate and up-to-date.
- Select Primary Sampling Units (PSUs): Use your chosen sampling method (e.g., random, systematic) to select the first set of larger units. These should be representative of the broader population.
- Select Secondary Sampling Units (SSUs): Within each PSU, select smaller units using the same sampling method or a different one. This step further narrows down your sample.
- Continue with Further Stages if Needed: If your plan includes more stages, continue selecting smaller units within each SSU until you reach the ultimate sampling units (USUs).
- Train Data Collectors: Ensure that all personnel involved in data collection are thoroughly trained in the sampling methods and protocols. This helps maintain consistency and accuracy.
- Conduct Pilot Testing: Before full implementation, conduct a pilot test to identify and address any potential issues in your sampling process. Adjust your plan as needed based on the results.
- Collect Data: Implement your sampling plan and collect data from the selected units. Follow established protocols to ensure data quality and integrity.
- Monitor Progress and Quality Control: Continuously monitor the data collection process to ensure it stays on track. Implement quality control measures to address any issues promptly.
- Analyze Data: Use appropriate statistical techniques to analyze the collected data, ensuring your findings are representative and reliable.
- Report Findings: Compile and report your findings, providing insights and recommendations based on your research objectives.
Sampling Tools and Software
Using the right tools and software is crucial to implement multistage sampling effectively. These tools can assist in various aspects of the process, from designing the sampling plan to collecting and analyzing the data. Some of the most valuable tools and software for sampling include:
- Statistical Software Packages: Software such as SPSS, SAS, R, and Stata are invaluable for designing sampling plans, calculating sample sizes, and performing complex data analyses. These tools offer a wide range of statistical functions and are essential for managing large datasets.
- Sampling Design Software: Tools like PASS (Power Analysis and Sample Size) and G*Power help researchers determine the appropriate sample size and design their sampling plans effectively.
- Survey Management Software: Platforms like Appinio provide comprehensive solutions for designing surveys, managing data collection, and tracking responses. These tools are advantageous for organizing and implementing large-scale surveys.
- Project Management Software: Tools like Trello, Asana, and Microsoft Project can help manage the logistics of your sampling project, ensuring that all tasks are completed on time and resources are effectively allocated.
Multistage Sampling Examples
Understanding how multistage sampling works in real-world scenarios can help solidify your grasp of the method and its applications.
Public Health Studies
In public health research, multistage sampling is often used to conduct large-scale surveys that require representative data from diverse populations.
Scenario: A national health survey aims to assess the prevalence of diabetes among adults in the country.
Stages of Sampling:
- Primary Stage (PSUs): Select regions or states as primary sampling units. These regions are chosen based on demographic diversity and geographic distribution.
- Secondary Stage (SSUs): Within each selected region, randomly select districts or counties. This helps narrow down the large population into manageable sections.
- Tertiary Stage: Within each district, select communities or neighborhoods. This stage ensures that different localities within each district are represented.
- Final Stage (USUs): Finally, within each neighborhood, households are randomly selected, and then individual adults within those households are chosen to participate in the survey.
This multistage approach ensures that the sample is representative of the entire country's adult population, capturing regional, urban-rural, and socio-economic variations.
Market Research
In market research, multistage sampling helps companies understand consumer behavior and preferences across different regions and demographics.
Scenario: A company wants to gauge consumer satisfaction with a new product in various parts of the country.
Stages of Sampling:
- Primary Stage (PSUs): Select cities or metropolitan areas across the country. These areas are chosen to reflect different market sizes and economic conditions.
- Secondary Stage (SSUs): Select neighborhoods or districts within each city. This ensures a mix of urban, suburban, and possibly rural areas.
- Final Stage (USUs): Households are randomly chosen within each neighborhood. Specific individuals within those households are then selected to participate in the survey.
Using multistage sampling, the company can collect data that reflects a wide range of consumer experiences and preferences, helping them tailor their marketing strategies effectively.
Conclusion for Multistage Sampling
Multistage sampling is an invaluable tool in the researcher's toolkit, offering a structured yet flexible approach to studying large and diverse populations. By breaking the sampling process into multiple stages, researchers can effectively manage logistical challenges and ensure that their samples are both representative and accurate. This guide has walked you through the fundamental concepts, advantages, and detailed steps involved in multistage sampling, providing you with an understanding of how to design and implement this method. With its ability to balance practicality with precision, multistage sampling stands out as a robust method suitable for a wide range of research fields, from public health to social sciences.
As you move forward with your research, remember that the key to successful multistage sampling lies in careful planning and thoughtful execution. Defining your population clearly, choosing appropriate sampling units, and utilizing the right tools and software are all crucial steps that will determine the quality of your findings. By adhering to the principles and best practices outlined in this guide, you can confidently apply multistage sampling to gather reliable data and derive meaningful insights. Whether you're conducting a small-scale study or an extensive national survey, the strategies you've learned here will help you navigate the complexities of multistage sampling, ensuring your research is effective and impactful.
How to Conduct Multistage Sampling in Minutes?
Appinio revolutionizes how companies conduct market research, making implementing complex methods like multistage sampling easy. As a real-time market research platform, Appinio empowers businesses to gain immediate consumer insights, driving fast, data-driven decisions without the hassle of traditional research processes.
By handling all the technical and research-heavy tasks, Appinio allows companies to focus on what truly matters – using real-time data to enhance their strategies and make informed decisions. Our platform is designed to be exciting, intuitive, and seamlessly integrated into everyday business operations, debunking the myth that market research is tedious or intimidating.
- Rapid Insights: Appinio's platform delivers from questions to insights in under 23 minutes for 1,000 respondents, ensuring businesses get the answers they need, fast.
- Ease of Use: With an intuitive interface, anyone can conduct market research without needing a PhD, making sophisticated methods like multistage sampling accessible to all.
- Global Reach: Companies can define their target group from over 1,200 characteristics and survey audiences in more than 90 countries, ensuring comprehensive and representative data.
Get facts and figures ðŸ§
Want to see more data insights? Our free reports are just the right thing for you!