What is Systematic Sampling? Definition, Types, Examples
Appinio Research · 28.05.2024 · 32min read
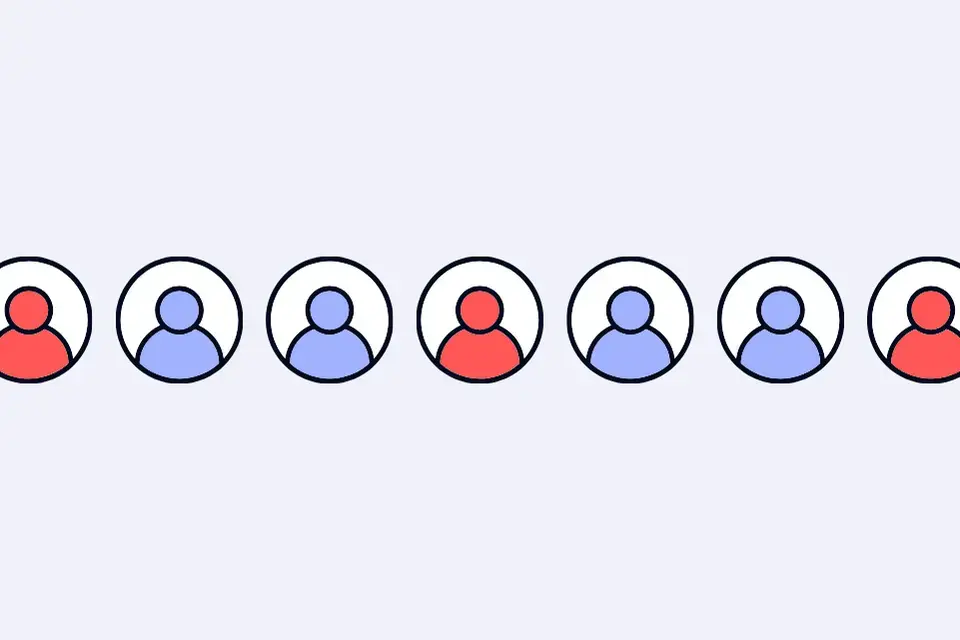
Content
Ever wondered how researchers select a group of people or items to study from a larger population? Well, that's where systematic sampling comes into play! Picture this: you have a massive pool of data or a large group of individuals, and you need to gather insights or draw conclusions from this vast sea of information.
Systematic sampling is like having a strategic plan to pick out just the right pieces from the puzzle without feeling overwhelmed. It's like having a methodical way of selecting every "nth" element, where "n" is the sampling interval, ensuring that each piece gets a fair chance to be part of the sample.
But why is systematic sampling so important? Because it's like having a smart shortcut – it lets you capture the essence of the entire population without having to scrutinize every single element. Plus, it maintains a good balance between randomness and efficiency, giving you reliable results without breaking a sweat.
What is Systematic Sampling?
Systematic sampling is a statistical sampling technique used to select a sample from a larger population in a systematic way. Unlike random sampling methods that select elements randomly, systematic sampling involves choosing every "kth" element from a list or population, where "k" represents the sampling interval. This method ensures that each member of the population has an equal chance of being included in the sample, provided that the sampling interval is chosen appropriately.
How Systematic Sampling Works
The process of systematic sampling involves several key steps:
- Define the Population: Identify the population of interest that you want to study or draw conclusions about.
- Determine the Sampling Interval: Decide on the sampling interval "k," which represents the number of elements or units between each selected sample.
- Randomly Select a Starting Point: Randomly choose a starting point within the population to initiate the sampling process.
- Select Sample Units at Regular Intervals: Select sample units at regular intervals based on the sampling interval "k." For example, if the sampling interval is 5, you would select every 5th element from the population list.
- Calculate the Sample Size: Calculate the sample size based on the desired level of precision and confidence. The sample size is determined by dividing the population size by the sampling interval.
By following these steps, researchers can ensure that their systematic sampling process is conducted effectively and yields reliable results. Systematic sampling provides a structured and efficient approach to sample selection, making it a valuable tool for researchers in various fields of study.
Understanding Systematic Sampling
Let's delve deeper into the intricacies of systematic sampling and understand its key components and implications.
What is a Sampling Frame?
A sampling frame is essentially the backbone of any sampling method, including systematic sampling. It represents the list or set of individuals or items from which you draw your sample. In systematic sampling, the sampling frame plays a critical role in ensuring the representativeness of your sample. It should ideally encompass the entire population you're interested in studying. For instance, if you're conducting a survey on customer satisfaction in a particular city, your sampling frame would consist of all the residents or households in that city.
Advantages of Systematic Sampling
Systematic sampling offers several advantages that make it a popular choice in research methodology:
- Efficiency: Systematic sampling allows researchers to cover a large portion of the population with minimal resources and time. By selecting every "kth" element from the sampling frame, you achieve a representative sample without having to assess every single unit in the population.
- Ease of Implementation: Unlike some complex sampling methods, systematic sampling is relatively straightforward to implement. Once you determine the sampling interval and select a starting point, the rest of the process follows a systematic approach, making it accessible to researchers with varying levels of expertise.
- Randomness: Despite its systematic nature, systematic sampling maintains a level of randomness, which is crucial for reducing sampling bias in the sample selection process. As long as the sampling frame is representative of the population, systematic sampling ensures that each member of the population has an equal chance of being included in the sample.
Disadvantages of Systematic Sampling
While systematic sampling offers many benefits, it's essential to acknowledge its limitations and potential drawbacks:
- Risk of Bias: Systematic sampling may introduce bias if the population has a hidden pattern or periodicity that aligns with the sampling interval. For example, if you're surveying employees' satisfaction in a company where shifts are assigned systematically, systematic sampling may inadvertently skew the results.
- Limited Representativeness: The representativeness of the sample in systematic sampling hinges on the randomness of the selection process and the adequacy of the sampling frame. If the sampling interval isn't chosen carefully or certain population segments are inadvertently excluded from the sampling frame, the sample may not accurately reflect the population's characteristics.
- Vulnerability to Errors: Errors in the sampling frame or selection process can undermine the validity and reliability of the results obtained through systematic sampling. It's crucial to validate the sampling frame and ensure the selection process follows the intended systematic approach to minimize errors.
Types of Systematic Sampling
Systematic sampling can be implemented in various ways, each tailored to different research objectives and population characteristics. Let's explore some common types of systematic sampling:
1. Linear Systematic Sampling
Linear systematic sampling involves selecting sample units at regular intervals along a linear or sequential order within the population.
Application: Linear systematic sampling is often used when the population is arranged in a sequential or linear manner, such as a queue, a production line, or a time series data.
Example: In a manufacturing plant with products moving along a conveyor belt, every 10th product could be systematically sampled for quality control inspection.
2. Randomized Systematic Sampling
Randomized systematic sampling combines elements of random sampling and systematic sampling by introducing randomness in the selection of the starting point.
Application: Randomized systematic sampling is useful when there is concern about potential biases introduced by the systematic selection process.
Example: In a population of students listed alphabetically, instead of starting with the first student, a random starting point is selected to reduce the risk of bias.
3. Circular Systematic Sampling
Circular systematic sampling involves selecting sample units within the population at regular intervals in a circular or cyclic manner.
Application: Circular systematic sampling is suitable for populations arranged in a circular or cyclic fashion, such as days of the week, months of the year, or compass directions.
Example: To study weekly fluctuations in sales, a researcher might systematically sample sales data every Monday as a starting point and then select data points at weekly intervals.
4. Multi-Stage Systematic Sampling
Multi-stage systematic sampling involves applying systematic sampling at multiple stages or levels within a population.
Application: Multi-stage systematic sampling is used when the population can be hierarchically divided into subgroups, with systematic sampling applied within each subgroup.
Example: In a national survey, states could be systematically sampled first, followed by systematic sampling within each selected state to obtain a representative sample.
5. Stratified Systematic Sampling
Stratified systematic sampling involves dividing the population into distinct strata based on specific characteristics and then applying systematic sampling within each stratum.
Application: Stratified systematic sampling ensures proportional representation of different subgroups within the population, leading to more accurate estimates for each subgroup.
Example: In a survey on household income, households could be stratified by income levels, and systematic sampling could be applied within each income stratum to ensure representation across all income groups.
By understanding the various types of systematic sampling and their applications, researchers can choose the most suitable approach to obtain representative samples for their research studies. Each type of systematic sampling offers unique advantages and considerations, allowing researchers to tailor their sampling strategy to the specific characteristics of the population and research objectives.
Systematic Sampling vs. Other Sampling Methods
When it comes to sampling methods, systematic sampling is just one of several options available. Let's explore how it compares to other commonly used sampling techniques:
Systematic Sampling vs. Random Sampling
Random sampling involves selecting individuals or items from a population at random, where each member has an equal chance of being chosen.
- Randomness: While systematic sampling introduces a level of systematicity by selecting every "kth" element, random sampling ensures complete randomness in selection.
- Representation: Random sampling may result in a more representative sample if executed properly, as it minimizes the risk of research bias.
- Efficiency: Systematic sampling is often more efficient than random sampling in terms of time and resources since it follows a systematic pattern.
Systematic Sampling vs. Stratified Sampling
Stratified sampling involves dividing the population into distinct subgroups or strata based on specific characteristics and then selecting samples from each stratum.
- Representation: Stratified sampling ensures that each population subgroup is represented proportionally in the sample, which can lead to more accurate estimates for each subgroup.
- Complexity: While systematic sampling is relatively straightforward, stratified sampling requires careful identification and stratification of subgroups, adding complexity to the sampling process.
- Precision: In cases where the population exhibits significant heterogeneity, stratified sampling may yield more precise estimates than systematic sampling.
Systematic Sampling vs. Cluster Sampling
Cluster sampling involves dividing the population into clusters or groups, selecting a random sample of clusters, and then sampling all members within the selected clusters.
- Cluster Size: In cluster sampling, the clusters themselves are the sampling units, whereas in systematic sampling, individual elements or units within the population are sampled directly.
- Efficiency: Cluster sampling can be more efficient when the population is naturally clustered or geographically dispersed, as it reduces the logistical challenges of reaching dispersed populations.
- Precision: Systematic sampling generally provides more precise estimates for individual elements or units within the population compared to cluster sampling, especially if the clusters are heterogeneous.
By understanding the strengths and weaknesses of different sampling methods, researchers can select the most appropriate approach based on their specific research objectives, the nature of the population, and the available resources. While systematic sampling offers efficiency and ease of implementation, other methods such as random sampling, stratified sampling, and cluster sampling provide alternative strategies for obtaining representative samples in diverse research contexts.
How to Conduct Systematic Sampling?
Now that we've grasped the fundamental concepts of systematic sampling, let's break down the process into actionable steps to guide you through its implementation.
1. Define the Population
The first step in conducting systematic sampling is to define the population you intend to study or draw conclusions about. The population represents the entire group or set of individuals or items you want to examine. It's crucial to define the population accurately to ensure that your sample is representative and applicable to the target population.
For example, if you're studying customer preferences for a new product, your population would consist of all potential customers who might purchase or use the product.
2. Determine the Sampling Interval
Once you've defined the population, the next step is to determine the sampling interval, denoted by "k." The sampling interval refers to the number of elements or units between each selected sample in the population. The choice of the sampling interval depends on various factors, including the size of the population, the desired level of precision, and the resources available for sampling.
It's essential to strike a balance between the sampling interval and the representativeness of the sample. A smaller sampling interval provides a more representative sample but may require more resources, whereas a larger sampling interval may be more efficient but could compromise the representativeness of the sample.
3. Randomly Select a Starting Point
After determining the sampling interval, you need to randomly select a starting point within the population. Random selection helps ensure that the sample is representative and reduces the risk of bias. You can use various methods to select a starting point randomly, such as using random number generators or randomly choosing a starting point from a predefined list. By introducing randomness in the selection process, you minimize the likelihood of systematic errors and ensure that each element in the population has an equal chance of being included in the sample.
4. Select Sample Units at Regular Intervals
Once you have a starting point, you can select sample units at regular intervals based on the sampling interval "k." For example, if the sampling interval is 5, you would select every 5th element from the population list. It's crucial to adhere strictly to the systematic pattern and avoid any deviations or biases in the selection process. By following a systematic approach, you maintain the randomness of the sample selection while ensuring that each element in the population has an equal chance of being included in the sample.
5. Calculate the Sample Size
Finally, calculate the sample size based on the desired level of precision and confidence. The sample size is determined by dividing the population size by the sampling interval. To calculate the sample size, divide the population size by the sampling interval. The formula is:
Sample size = Population size / k
Where:
- Sample size: The number of elements or units to be included in the sample.
- Population size: The total number of elements or units in the population.
- k: The sampling interval.
For example, if the population size is 1000 and the sampling interval is 10, the sample size would be:
Sample size = 1000 / 10 = 100
Calculating the sample size beforehand ensures that your sample is appropriately sized to yield statistically valid results. However, factors such as the margin of error, confidence level, and variability within the population must also be considered when determining the sample size. Striking the right balance between sample size and statistical precision is crucial for obtaining reliable and meaningful insights from systematic sampling. Alternatively, you can use our sample size calculator to easily determine your sample size.
In systematic sampling, defining your target audience with precision is critical to obtaining meaningful insights. However, the process can be time-consuming and complex. That's where platforms like Appinio come into play. With Appinio, you can easily define your exact audience, selecting from a wide range of characteristics and demographics.
Appinio takes care of all the heavy lifting, ensuring that your sample is representative and applicable to your target population. Say goodbye to the complexities of sampling and data collection – with Appinio, you can collect the data you need in minutes, allowing you to focus on making informed decisions for your business.
Ready to experience the power of Appinio? Book a demo today and see how it can transform your market research efforts.
Systematic Sampling Examples
Let's explore some examples to illustrate how systematic sampling works in real-world scenarios.
Example 1: Customer Satisfaction Survey
Imagine you're tasked with conducting a customer satisfaction survey for a retail store chain with 1000 customers. To obtain a representative sample using systematic sampling, follow these steps:
- Define the Population: The population consists of all 1000 customers who have made purchases at the retail store.
- Determine the Sampling Interval: Suppose you want to survey 100 customers. Calculate the sampling interval (𝑘):
𝑘 = Population size / Sample size = 1000 / 100 = 10 - Randomly Select a Starting Point: Randomly choose a customer from 1 to 10 as the starting point. Let's say you select customer number 3.
- Select Sample Units at Regular Intervals: Starting from customer number 3, select every 10th customer as part of the sample. The selected customers would be 3, 13, 23, 33, ..., 993.
Example 2: Quality Control Inspection
Suppose you're responsible for conducting quality control inspections on a production line that produces 5000 units per day. To ensure product quality using systematic sampling, follow these steps:
- Define the Population: The population consists of all 5000 units produced each day on the production line.
- Determine the Sampling Interval: Let's say you want to inspect 500 units per day. Calculate the sampling interval (𝑘):
𝑘 = Population size / Sample size = 5000 / 500 = 10 - Randomly Select a Starting Point: Randomly choose a unit from 1 to 10 as the starting point. Suppose you select unit number 7.
- Select Sample Units at Regular Intervals: Starting from unit number 7, select every 10th unit for inspection. The selected units would be 7, 17, 27, 37, ..., 4997.
These examples demonstrate how systematic sampling can be applied in various contexts to obtain representative samples efficiently. By following the systematic approach and applying appropriate calculations, researchers and professionals can make informed decisions based on the insights derived from the sampled data.
Systematic Sampling Considerations
Now that we've covered the basic steps of conducting systematic sampling, let's delve into some practical considerations that can enhance the reliability and validity of your sampling process.
Ensuring Randomness in Selecting the Starting Point
Randomness in selecting the starting point is crucial to minimize bias and ensure the representativeness of the sample. There are several methods you can employ to ensure randomness:
- Random Number Generators: Utilize random number generators, whether computer-based or manual, to select a starting point. This method eliminates human bias and ensures randomness in the selection process.
- Random Selection from a Predefined List: If your sampling frame is predefined (e.g., a list of addresses or customer IDs), randomly select a starting point from this list. Ensure that each element in the list has an equal chance of being chosen as the starting point.
- Shuffle and Pick: If you're working with physical samples or items, shuffle the list or items and blindly pick a starting point. This method introduces randomness and reduces the likelihood of systematic biases.
By incorporating randomness into the starting point selection, you increase the likelihood of obtaining a representative sample that accurately reflects the population's characteristics.
Dealing with Periodicity
Periodicity refers to the presence of a repeating pattern or cycle in the population that aligns with the sampling interval. To mitigate the effects of periodicity on systematic sampling:
- Introduce Randomness: If the population exhibits periodic patterns, introduce randomness in the selection process to disrupt the pattern. For example, randomly select the starting point or apply a random offset to the sampling interval.
- Adjust Sampling Interval: If possible, adjust the sampling interval to avoid aligning with the periodic pattern. Experiment with different intervals to minimize the impact of periodicity on the sample selection process.
- Combine with Other Sampling Methods: Consider combining systematic sampling with other sampling methods, such as stratified sampling or cluster sampling, to overcome the limitations imposed by periodicity. By diversifying your sampling approach, you can obtain a more robust and representative sample.
Handling Non-Random Sampling Frames
In some cases, the sampling frame may not be entirely random, leading to potential biases in the sample selection process. To address this challenge:
- Evaluate Sampling Frame: Thoroughly evaluate the sampling frame to identify any inherent biases or limitations. Assess its representativeness and consider whether adjustments are necessary to improve its validity.
- Correct Biases: If biases are present in the sampling frame, implement corrective measures to mitigate their impact on the sample selection process. For example, adjust the sample weights or apply statistical techniques to account for non-randomness in the sampling frame.
- Expand Sampling Frame: If possible, expand the sampling frame to include a more diverse and representative range of elements or units. By broadening the scope of the sampling frame, you can reduce the risk of bias and enhance the generalizability of the findings.
Adjustments for Skewed Distributions
In cases where the population exhibits a skewed distribution, systematic sampling may yield a sample that does not accurately reflect the population's characteristics. To address this issue:
- Stratified Sampling: If the population is heterogeneous and exhibits significant variability, consider stratified sampling. Divide the population into homogeneous strata based on relevant characteristics and then apply systematic sampling within each stratum. This approach ensures that each subgroup is adequately represented in the sample.
- Weighting: Apply weighting techniques to adjust for the unequal probabilities of selection introduced by systematic sampling. Assign weights to each sampled unit based on its probability of selection, ensuring that units with lower probabilities are given greater weight in the analysis.
- Alternative Sampling Methods: Explore alternative sampling methods, such as cluster sampling or quota sampling, that may be more suitable for skewed distributions. These methods offer flexibility in sample selection and can accommodate non-normal distributions more effectively than systematic sampling alone.
How to Analyze Systematic Sampling Results?
Now that you've completed the systematic sampling process and collected your data, it's time to analyze the results. We'll explore various techniques for analyzing systematic sampling data and extracting meaningful insights.
1. Estimate Population Parameters
One of the primary objectives of systematic sampling is to estimate population parameters based on the characteristics of the sample. Population parameters, such as the mean, median, variance, or proportion, provide valuable insights into the population's characteristics.
To estimate population parameters from your sample data, you can use statistical techniques such as:
- Point Estimation: Calculate point estimates of population parameters using sample statistics. For example, use the sample mean to estimate the population mean or the sample proportion to estimate the population proportion.
- Interval Estimation: Construct confidence intervals around the point estimates to quantify the uncertainty associated with the estimates. Confidence intervals provide a range of values within which the population parameter is likely to fall with a certain level of confidence.
Estimating population parameters from your systematic sample allows you to make inferences about the population with a known degree of uncertainty.
2. Calculate the Standard Error
Standard error measures the variability or uncertainty in estimating a population parameter based on a sample. It provides a measure of the precision of the estimate and helps assess the reliability of the results.
To calculate the standard error for systematic sampling, you can use the following formula:
SE = s / √n
Where:
- SE is the standard error.
- s is the sample standard deviation.
- n is the sample size.
For example, if you have a sample with a standard deviation of 2 and a sample size of 100, the standard error would be:
SE = 2 / √100 = 0.2
The standard error quantifies the dispersion of sample estimates around the true population parameter. A smaller standard error indicates greater precision in the estimate, while a larger standard error suggests greater uncertainty.
3. Interpret Confidence Intervals
Confidence intervals provide a range of values within which the population parameter is likely to fall with a certain level of confidence. The width of the confidence interval reflects the precision of the estimate, with narrower intervals indicating greater precision. When interpreting confidence intervals, consider the following:
- Confidence Level: The confidence level represents the probability that the true population parameter falls within the confidence interval. Standard confidence levels include 90%, 95%, and 99%.
- Margin of Error: The margin of error, or the half-width of the confidence interval, quantifies the uncertainty associated with the estimate. A smaller margin of error indicates greater precision in the estimate.
Interpreting confidence intervals allows you to make informed decisions and draw meaningful conclusions about the population based on your sample data.
4. Assess Sampling Bias
Sampling bias occurs when specific segments of the population are systematically excluded or underrepresented in the sample, leading to skewed or inaccurate estimates of population parameters. To assess sampling bias in systematic sampling:
- Compare Sample Characteristics: Compare the characteristics of the sample with those of the population to identify any discrepancies or biases. Look for systematic patterns or differences that may indicate sampling bias.
- Sensitivity Analysis: Conduct sensitivity analysis using varying key parameters, such as the sampling interval or starting point, to assess the robustness of the results. Evaluate how changes in these parameters affect the estimates of population parameters.
- Bias Correction: If sampling bias is detected, consider applying bias correction techniques to adjust the estimates and improve their accuracy. These techniques may involve weighting adjustments or statistical modeling to account for the biases introduced by systematic sampling.
By assessing sampling bias and addressing any discrepancies or limitations in the sampling process, you can enhance the validity and reliability of your systematic sampling results.
Systematic Sampling Tips
Implementing systematic sampling effectively requires careful planning and attention to detail. Here are some best practices and tips to enhance the quality and reliability of your sampling process:
- Ensure Adequate Sampling Frame: Start with a comprehensive and representative sampling frame that includes all elements or units of the population of interest. A well-defined sampling frame forms the basis for systematic sampling and ensures the generalizability of your results.
- Randomize Starting Point Selection: To minimize bias and ensure randomness in the sample selection process, randomly select the starting point within the sampling frame. Utilize random number generators or random selection techniques to achieve randomness.
- Verify Sampling Interval Appropriateness: Evaluate the sampling interval to ensure it strikes a balance between efficiency and representativeness. Adjust the sampling interval as needed to avoid periodicity or bias in the sample selection process.
- Document Sampling Procedure: Maintain detailed documentation of the sampling procedure, including the sampling frame, sampling interval, starting point selection method, and any adjustments made during the sampling process. Documentation ensures transparency and reproducibility in your research.
- Validate Sample Representativeness: Assess the representativeness of the sample by comparing its characteristics with those of the population. Conduct sensitivity analyses and validity checks to verify the robustness of your sample.
- Consider Stratification: If the population exhibits significant heterogeneity, consider stratified sampling to ensure adequate representation of different subgroups. Stratification improves the precision of estimates and enhances the validity of the sample.
- Monitor Sampling Errors: Regularly monitor and address potential sources of sampling errors, such as biases, non-response, or data collection inconsistencies. Implement quality control measures to minimize errors and ensure the reliability of your results.
- Calculate Sample Size Appropriately: Determine the sample size based on statistical principles and considerations such as the desired level of precision, confidence level, and variability within the population. Use sample size calculators or statistical software to determine the optimal sample size.
- Apply Weighting Techniques: If necessary, apply weighting techniques to adjust for unequal probabilities of selection or non-response in the sample. Weighted estimates help correct biases and improve the accuracy of population parameter estimates.
- Validate Results with External Data: Validate your systematic sampling results by comparing them with external data sources or alternative sampling methods. Cross-validation enhances the credibility of your findings and provides additional confidence in the results.
By following these tips, you can optimize your systematic sampling process and obtain reliable, actionable insights from your research.
Conclusion for Systematic Sampling
Systematic sampling offers a straightforward yet powerful approach to gathering data from large populations. By following a systematic pattern of selection, researchers can efficiently capture the essence of the entire population without having to examine every single element. This method not only saves time and resources but also ensures the validity and reliability of the results obtained. Whether you're conducting market research, opinion polling, or quality control, systematic sampling provides a reliable framework for obtaining meaningful insights.
Incorporating best practices such as randomizing the starting point selection, validating the representativeness of the sample, and assessing potential biases can further enhance the quality of systematic sampling outcomes. By implementing these strategies and techniques, researchers can confidently draw conclusions and make informed decisions based on the findings obtained through systematic sampling. So, the next time you need to gather data from a large population, remember the power of systematic sampling – it's your trusted companion in the journey of research and discovery.
How to Conduct Sampling in Minutes?
Looking for an even better and easier alternative to systematic sampling? Enter Appinio, the real-time market research platform revolutionizing how companies gather consumer insights. With Appinio, conducting your own market research is not only fast but also seamless. Let Appinio take care of the heavy lifting in research and tech so you can focus on what matters – making informed decisions that drive your business forward.
Here's why you should give it a try:
- From Questions to Insights in Minutes: With Appinio, there's no waiting around for results. Get actionable insights in minutes, allowing you to make faster data-driven decisions for your business.
- Intuitive Platform for Everyone: No need for a PhD in research. Our platform is so intuitive that anyone can use it, making market research accessible to all.
- Define Your Audience Seamlessly: With over 1200 characteristics to choose from, Appinio allows you to precisely define your target group and survey them in over 90 countries. Say goodbye to guesswork and hello to targeted insights.
Get facts and figures 🧠
Want to see more data insights? Our free reports are just the right thing for you!