Causal Research: Definition, Design, Tips, Examples
Appinio Research · 21.02.2024 · 34min read
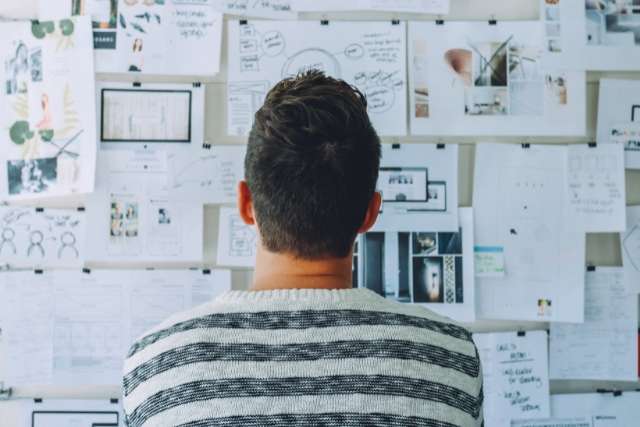
Content
Ever wondered why certain events lead to specific outcomes? Understanding causality—the relationship between cause and effect—is crucial for unraveling the mysteries of the world around us.
In this guide on causal research, we delve into the methods, techniques, and principles behind identifying and establishing cause-and-effect relationships between variables. Whether you're a seasoned researcher or new to the field, this guide will equip you with the knowledge and tools to conduct rigorous causal research and draw meaningful conclusions that can inform decision-making and drive positive change.
What is Causal Research?
Causal research is a methodological approach used in scientific inquiry to investigate cause-and-effect relationships between variables. Unlike correlational or descriptive research, which merely examine associations or describe phenomena, causal research aims to determine whether changes in one variable cause changes in another variable.
Importance of Causal Research
Understanding the importance of causal research is crucial for appreciating its role in advancing knowledge and informing decision-making across various fields. Here are key reasons why causal research is significant:
- Establishing Causality: Causal research enables researchers to determine whether changes in one variable directly cause changes in another variable. This helps identify effective interventions, predict outcomes, and inform evidence-based practices.
- Guiding Policy and Practice: By identifying causal relationships, causal research provides empirical evidence to support policy decisions, program interventions, and business strategies. Decision-makers can use causal findings to allocate resources effectively and address societal challenges.
- Informing Predictive Modeling: Causal research contributes to the development of predictive models by elucidating causal mechanisms underlying observed phenomena. Predictive models based on causal relationships can accurately forecast future outcomes and trends.
- Advancing Scientific Knowledge: Causal research contributes to the cumulative body of scientific knowledge by testing hypotheses, refining theories, and uncovering underlying mechanisms of phenomena. It fosters a deeper understanding of complex systems and phenomena.
- Mitigating Confounding Factors: Understanding causal relationships allows researchers to control for confounding variables and reduce bias in their studies. By isolating the effects of specific variables, researchers can draw more valid and reliable conclusions.
Causal Research Distinction from Other Research
Understanding the distinctions between causal research and other types of research methodologies is essential for researchers to choose the most appropriate approach for their study objectives. Let's explore the differences and similarities between causal research and descriptive, exploratory, and correlational research methodologies.
Descriptive vs. Causal Research
Descriptive research focuses on describing characteristics, behaviors, or phenomena without manipulating variables or establishing causal relationships. It provides a snapshot of the current state of affairs but does not attempt to explain why certain phenomena occur.
Causal research, on the other hand, seeks to identify cause-and-effect relationships between variables by systematically manipulating independent variables and observing their effects on dependent variables. Unlike descriptive research, causal research aims to determine whether changes in one variable directly cause changes in another variable.
Similarities:
- Both descriptive and causal research involve empirical observation and data collection.
- Both types of research contribute to the scientific understanding of phenomena, albeit through different approaches.
Differences:
- Descriptive research focuses on describing phenomena, while causal research aims to explain why phenomena occur by identifying causal relationships.
- Descriptive research typically uses observational methods, while causal research often involves experimental designs or causal inference techniques to establish causality.
Exploratory vs. Causal Research
Exploratory research aims to explore new topics, generate hypotheses, or gain initial insights into phenomena. It is often conducted when little is known about a subject and seeks to generate ideas for further investigation.
Causal research, on the other hand, is concerned with testing hypotheses and establishing cause-and-effect relationships between variables. It builds on existing knowledge and seeks to confirm or refute causal hypotheses through systematic investigation.
Similarities:
- Both exploratory and causal research contribute to the generation of knowledge and theory development.
- Both types of research involve systematic inquiry and data analysis to answer research questions.
Differences:
- Exploratory research focuses on generating hypotheses and exploring new areas of inquiry, while causal research aims to test hypotheses and establish causal relationships.
- Exploratory research is more flexible and open-ended, while causal research follows a more structured and hypothesis-driven approach.
Correlational vs. Causal Research
Correlational research examines the relationship between variables without implying causation. It identifies patterns of association or co-occurrence between variables but does not establish the direction or causality of the relationship.
Causal research, on the other hand, seeks to establish cause-and-effect relationships between variables by systematically manipulating independent variables and observing their effects on dependent variables. It goes beyond mere association to determine whether changes in one variable directly cause changes in another variable.
Similarities:
- Both correlational and causal research involve analyzing relationships between variables.
- Both types of research contribute to understanding the nature of associations between variables.
Differences:
- Correlational research focuses on identifying patterns of association, while causal research aims to establish causal relationships.
- Correlational research does not manipulate variables, while causal research involves systematically manipulating independent variables to observe their effects on dependent variables.
How to Formulate Causal Research Hypotheses?
Crafting research questions and hypotheses is the foundational step in any research endeavor. Defining your variables clearly and articulating the causal relationship you aim to investigate is essential. Let's explore this process further.
1. Identify Variables
Identifying variables involves recognizing the key factors you will manipulate or measure in your study. These variables can be classified into independent, dependent, and confounding variables.
- Independent Variable (IV): This is the variable you manipulate or control in your study. It is the presumed cause that you want to test.
- Dependent Variable (DV): The dependent variable is the outcome or response you measure. It is affected by changes in the independent variable.
- Confounding Variables: These are extraneous factors that may influence the relationship between the independent and dependent variables, leading to spurious correlations or erroneous causal inferences. Identifying and controlling for confounding variables is crucial for establishing valid causal relationships.
2. Establish Causality
Establishing causality requires meeting specific criteria outlined by scientific methodology. While correlation between variables may suggest a relationship, it does not imply causation. To establish causality, researchers must demonstrate the following:
- Temporal Precedence: The cause must precede the effect in time. In other words, changes in the independent variable must occur before changes in the dependent variable.
- Covariation of Cause and Effect: Changes in the independent variable should be accompanied by corresponding changes in the dependent variable. This demonstrates a consistent pattern of association between the two variables.
- Elimination of Alternative Explanations: Researchers must rule out other possible explanations for the observed relationship between variables. This involves controlling for confounding variables and conducting rigorous experimental designs to isolate the effects of the independent variable.
3. Write Clear and Testable Hypotheses
Hypotheses serve as tentative explanations for the relationship between variables and provide a framework for empirical testing. A well-formulated hypothesis should be:
- Specific: Clearly state the expected relationship between the independent and dependent variables.
- Testable: The hypothesis should be capable of being empirically tested through observation or experimentation.
- Falsifiable: There should be a possibility of proving the hypothesis false through empirical evidence.
For example, a hypothesis in a study examining the effect of exercise on weight loss could be: "Increasing levels of physical activity (IV) will lead to greater weight loss (DV) among participants (compared to those with lower levels of physical activity)."
By formulating clear hypotheses and operationalizing variables, researchers can systematically investigate causal relationships and contribute to the advancement of scientific knowledge.
Causal Research Design
Designing your research study involves making critical decisions about how you will collect and analyze data to investigate causal relationships.
Experimental vs. Observational Designs
One of the first decisions you'll make when designing a study is whether to employ an experimental or observational design. Each approach has its strengths and limitations, and the choice depends on factors such as the research question, feasibility, and ethical considerations.
- Experimental Design: In experimental designs, researchers manipulate the independent variable and observe its effects on the dependent variable while controlling for confounding variables. Random assignment to experimental conditions allows for causal inferences to be drawn.
Example: A study testing the effectiveness of a new teaching method on student performance by randomly assigning students to either the experimental group (receiving the new teaching method) or the control group (receiving the traditional method). - Observational Design: Observational designs involve observing and measuring variables without intervention. Researchers may still examine relationships between variables but cannot establish causality as definitively as in experimental designs.
Example: A study observing the association between socioeconomic status and health outcomes by collecting data on income, education level, and health indicators from a sample of participants.
Control and Randomization
Control and randomization are crucial aspects of experimental design that help ensure the validity of causal inferences.
- Control: Controlling for extraneous variables involves holding constant factors that could influence the dependent variable, except for the independent variable under investigation. This helps isolate the effects of the independent variable.
Example: In a medication trial, controlling for factors such as age, gender, and pre-existing health conditions ensures that any observed differences in outcomes can be attributed to the medication rather than other variables. - Randomization: Random assignment of participants to experimental conditions helps distribute potential confounders evenly across groups, reducing the likelihood of systematic biases and allowing for causal conclusions.
Example: Randomly assigning patients to treatment and control groups in a clinical trial ensures that both groups are comparable in terms of baseline characteristics, minimizing the influence of extraneous variables on treatment outcomes.
Internal and External Validity
Two key concepts in research design are internal validity and external validity, which relate to the credibility and generalizability of study findings, respectively.
- Internal Validity: Internal validity refers to the extent to which the observed effects can be attributed to the manipulation of the independent variable rather than confounding factors. Experimental designs typically have higher internal validity due to their control over extraneous variables.
Example: A study examining the impact of a training program on employee productivity would have high internal validity if it could confidently attribute changes in productivity to the training intervention. - External Validity: External validity concerns the extent to which study findings can be generalized to other populations, settings, or contexts. While experimental designs prioritize internal validity, they may sacrifice external validity by using highly controlled conditions that do not reflect real-world scenarios.
Example: Findings from a laboratory study on memory retention may have limited external validity if the experimental tasks and conditions differ significantly from real-life learning environments.
Types of Experimental Designs
Several types of experimental designs are commonly used in causal research, each with its own strengths and applications.
- Randomized Control Trials (RCTs): RCTs are considered the gold standard for assessing causality in research. Participants are randomly assigned to experimental and control groups, allowing researchers to make causal inferences.
Example: A pharmaceutical company testing a new drug's efficacy would use an RCT to compare outcomes between participants receiving the drug and those receiving a placebo. - Quasi-Experimental Designs: Quasi-experimental designs lack random assignment but still attempt to establish causality by controlling for confounding variables through design or statistical analysis.
Example: A study evaluating the effectiveness of a smoking cessation program might compare outcomes between participants who voluntarily enroll in the program and a matched control group of non-enrollees.
By carefully selecting an appropriate research design and addressing considerations such as control, randomization, and validity, researchers can conduct studies that yield credible evidence of causal relationships and contribute valuable insights to their field of inquiry.
Causal Research Data Collection
Collecting data is a critical step in any research study, and the quality of the data directly impacts the validity and reliability of your findings.
Choosing Measurement Instruments
Selecting appropriate measurement instruments is essential for accurately capturing the variables of interest in your study. The choice of measurement instrument depends on factors such as the nature of the variables, the target population, and the research objectives.
- Surveys: Surveys are commonly used to collect self-reported data on attitudes, opinions, behaviors, and demographics. They can be administered through various methods, including paper-and-pencil surveys, online surveys, and telephone interviews.
- Observations: Observational methods involve systematically recording behaviors, events, or phenomena as they occur in natural settings. Observations can be structured (following a predetermined checklist) or unstructured (allowing for flexible data collection).
- Psychological Tests: Psychological tests are standardized instruments designed to measure specific psychological constructs, such as intelligence, personality traits, or emotional functioning. These tests often have established reliability and validity.
- Physiological Measures: Physiological measures, such as heart rate, blood pressure, or brain activity, provide objective data on bodily processes. They are commonly used in health-related research but require specialized equipment and expertise.
- Existing Databases: Researchers may also utilize existing datasets, such as government surveys, public health records, or organizational databases, to answer research questions. Secondary data analysis can be cost-effective and time-saving but may be limited by the availability and quality of data.
Ensuring accurate data collection is the cornerstone of any successful research endeavor. With the right tools in place, you can unlock invaluable insights to drive your causal research forward. From surveys to tests, each instrument offers a unique lens through which to explore your variables of interest.
At Appinio, we understand the importance of robust data collection methods in informing impactful decisions. Let us empower your research journey with our intuitive platform, where you can effortlessly gather real-time consumer insights to fuel your next breakthrough.
Ready to take your research to the next level? Book a demo today and see how Appinio can revolutionize your approach to data collection!
Sampling Techniques
Sampling involves selecting a subset of individuals or units from a larger population to participate in the study. The goal of sampling is to obtain a representative sample that accurately reflects the characteristics of the population of interest.
- Probability Sampling: Probability sampling methods involve randomly selecting participants from the population, ensuring that each member of the population has an equal chance of being included in the sample. Common probability sampling techniques include simple random sampling, stratified sampling, and cluster sampling.
- Non-Probability Sampling: Non-probability sampling methods do not involve random selection and may introduce biases into the sample. Examples of non-probability sampling techniques include convenience sampling, purposive sampling, and snowball sampling.
The choice of sampling technique depends on factors such as the research objectives, population characteristics, resources available, and practical constraints. Researchers should strive to minimize sampling bias and maximize the representativeness of the sample to enhance the generalizability of their findings.
Ethical Considerations
Ethical considerations are paramount in research and involve ensuring the rights, dignity, and well-being of research participants. Researchers must adhere to ethical principles and guidelines established by professional associations and institutional review boards (IRBs).
- Informed Consent: Participants should be fully informed about the nature and purpose of the study, potential risks and benefits, their rights as participants, and any confidentiality measures in place. Informed consent should be obtained voluntarily and without coercion.
- Privacy and Confidentiality: Researchers should take steps to protect the privacy and confidentiality of participants' personal information. This may involve anonymizing data, securing data storage, and limiting access to identifiable information.
- Minimizing Harm: Researchers should mitigate any potential physical, psychological, or social harm to participants. This may involve conducting risk assessments, providing appropriate support services, and debriefing participants after the study.
- Respect for Participants: Researchers should respect participants' autonomy, diversity, and cultural values. They should seek to foster a trusting and respectful relationship with participants throughout the research process.
- Publication and Dissemination: Researchers have a responsibility to accurately report their findings and acknowledge contributions from participants and collaborators. They should adhere to principles of academic integrity and transparency in disseminating research results.
By addressing ethical considerations in research design and conduct, researchers can uphold the integrity of their work, maintain trust with participants and the broader community, and contribute to the responsible advancement of knowledge in their field.
Causal Research Data Analysis
Once data is collected, it must be analyzed to draw meaningful conclusions and assess causal relationships.
Causal Inference Methods
Causal inference methods are statistical techniques used to identify and quantify causal relationships between variables in observational data. While experimental designs provide the most robust evidence for causality, observational studies often require more sophisticated methods to account for confounding factors.
- Difference-in-Differences (DiD): DiD compares changes in outcomes before and after an intervention between a treatment group and a control group, controlling for pre-existing trends. It estimates the average treatment effect by differencing the changes in outcomes between the two groups over time.
- Instrumental Variables (IV): IV analysis relies on instrumental variables—variables that affect the treatment variable but not the outcome—to estimate causal effects in the presence of endogeneity. IVs should be correlated with the treatment but uncorrelated with the error term in the outcome equation.
- Regression Discontinuity (RD): RD designs exploit naturally occurring thresholds or cutoff points to estimate causal effects near the threshold. Participants just above and below the threshold are compared, assuming that they are similar except for their proximity to the threshold.
- Propensity Score Matching (PSM): PSM matches individuals or units based on their propensity scores—the likelihood of receiving the treatment—creating comparable groups with similar observed characteristics. Matching reduces selection bias and allows for causal inference in observational studies.
Assessing Causality Strength
Assessing the strength of causality involves determining the magnitude and direction of causal effects between variables. While statistical significance indicates whether an observed relationship is unlikely to occur by chance, it does not necessarily imply a strong or meaningful effect.
- Effect Size: Effect size measures the magnitude of the relationship between variables, providing information about the practical significance of the results. Standard effect size measures include Cohen's d for mean differences and odds ratios for categorical outcomes.
- Confidence Intervals: Confidence intervals provide a range of values within which the actual effect size is likely to lie with a certain degree of certainty. Narrow confidence intervals indicate greater precision in estimating the true effect size.
- Practical Significance: Practical significance considers whether the observed effect is meaningful or relevant in real-world terms. Researchers should interpret results in the context of their field and the implications for stakeholders.
Handling Confounding Variables
Confounding variables are extraneous factors that may distort the observed relationship between the independent and dependent variables, leading to spurious or biased conclusions. Addressing confounding variables is essential for establishing valid causal inferences.
- Statistical Control: Statistical control involves including confounding variables as covariates in regression models to partially out their effects on the outcome variable. Controlling for confounders reduces bias and strengthens the validity of causal inferences.
- Matching: Matching participants or units based on observed characteristics helps create comparable groups with similar distributions of confounding variables. Matching reduces selection bias and mimics the randomization process in experimental designs.
- Sensitivity Analysis: Sensitivity analysis assesses the robustness of study findings to changes in model specifications or assumptions. By varying analytical choices and examining their impact on results, researchers can identify potential sources of bias and evaluate the stability of causal estimates.
- Subgroup Analysis: Subgroup analysis explores whether the relationship between variables differs across subgroups defined by specific characteristics. Identifying effect modifiers helps understand the conditions under which causal effects may vary.
By employing rigorous causal inference methods, assessing the strength of causality, and addressing confounding variables, researchers can confidently draw valid conclusions about causal relationships in their studies, advancing scientific knowledge and informing evidence-based decision-making.
Causal Research Examples
Examples play a crucial role in understanding the application of causal research methods and their impact across various domains. Let's explore some detailed examples to illustrate how causal research is conducted and its real-world implications:
Example 1: Software as a Service (SaaS) User Retention Analysis
Suppose a SaaS company wants to understand the factors influencing user retention and engagement with their platform. The company conducts a longitudinal observational study, collecting data on user interactions, feature usage, and demographic information over several months.
- Design: The company employs an observational cohort study design, tracking cohorts of users over time to observe changes in retention and engagement metrics. They use analytics tools to collect data on user behavior, such as logins, feature usage, session duration, and customer support interactions.
- Data Collection: Data is collected from the company's platform logs, customer relationship management (CRM) system, and user surveys. Key metrics include user churn rates, active user counts, feature adoption rates, and Net Promoter Scores (NPS).
- Analysis: Using statistical techniques like survival analysis and regression modeling, the company identifies factors associated with user retention, such as feature usage patterns, onboarding experiences, customer support interactions, and subscription plan types.
- Findings: The analysis reveals that users who engage with specific features early in their lifecycle have higher retention rates, while those who encounter usability issues or lack personalized onboarding experiences are more likely to churn. The company uses these insights to optimize product features, improve onboarding processes, and enhance customer support strategies to increase user retention and satisfaction.
Example 2: Business Impact of Digital Marketing Campaign
Consider a technology startup launching a digital marketing campaign to promote its new product offering. The company conducts an experimental study to evaluate the effectiveness of different marketing channels in driving website traffic, lead generation, and sales conversions.
- Design: The company implements an A/B testing design, randomly assigning website visitors to different marketing treatment conditions, such as Google Ads, social media ads, email campaigns, or content marketing efforts. They track user interactions and conversion events using web analytics tools and marketing automation platforms.
- Data Collection: Data is collected on website traffic, click-through rates, conversion rates, lead generation, and sales revenue. The company also gathers demographic information and user feedback through surveys and customer interviews to understand the impact of marketing messages and campaign creatives.
- Analysis: Utilizing statistical methods like hypothesis testing and multivariate analysis, the company compares key performance metrics across different marketing channels to assess their effectiveness in driving user engagement and conversion outcomes. They calculate return on investment (ROI) metrics to evaluate the cost-effectiveness of each marketing channel.
- Findings: The analysis reveals that social media ads outperform other marketing channels in generating website traffic and lead conversions, while email campaigns are more effective in nurturing leads and driving sales conversions. Armed with these insights, the company allocates marketing budgets strategically, focusing on channels that yield the highest ROI and adjusting messaging and targeting strategies to optimize campaign performance.
These examples demonstrate the diverse applications of causal research methods in addressing important questions, informing policy decisions, and improving outcomes in various fields. By carefully designing studies, collecting relevant data, employing appropriate analysis techniques, and interpreting findings rigorously, researchers can generate valuable insights into causal relationships and contribute to positive social change.
How to Interpret Causal Research Results?
Interpreting and reporting research findings is a crucial step in the scientific process, ensuring that results are accurately communicated and understood by stakeholders.
Interpreting Statistical Significance
Statistical significance indicates whether the observed results are unlikely to occur by chance alone, but it does not necessarily imply practical or substantive importance. Interpreting statistical significance involves understanding the meaning of p-values and confidence intervals and considering their implications for the research findings.
- P-values: A p-value represents the probability of obtaining the observed results (or more extreme results) if the null hypothesis is true. A p-value below a predetermined threshold (typically 0.05) suggests that the observed results are statistically significant, indicating that the null hypothesis can be rejected in favor of the alternative hypothesis.
- Confidence Intervals: Confidence intervals provide a range of values within which the true population parameter is likely to lie with a certain degree of confidence (e.g., 95%). If the confidence interval does not include the null value, it suggests that the observed effect is statistically significant at the specified confidence level.
Interpreting statistical significance requires considering factors such as sample size, effect size, and the practical relevance of the results rather than relying solely on p-values to draw conclusions.
Discussing Practical Significance
While statistical significance indicates whether an effect exists, practical significance evaluates the magnitude and meaningfulness of the effect in real-world terms. Discussing practical significance involves considering the relevance of the results to stakeholders and assessing their impact on decision-making and practice.
- Effect Size: Effect size measures the magnitude of the observed effect, providing information about its practical importance. Researchers should interpret effect sizes in the context of their field and the scale of measurement (e.g., small, medium, or large effect sizes).
- Contextual Relevance: Consider the implications of the results for stakeholders, policymakers, and practitioners. Are the observed effects meaningful in the context of existing knowledge, theory, or practical applications? How do the findings contribute to addressing real-world problems or informing decision-making?
Discussing practical significance helps contextualize research findings and guide their interpretation and application in practice, beyond statistical significance alone.
Addressing Limitations and Assumptions
No study is without limitations, and researchers should transparently acknowledge and address potential biases, constraints, and uncertainties in their research design and findings.
- Methodological Limitations: Identify any limitations in study design, data collection, or analysis that may affect the validity or generalizability of the results. For example, sampling biases, measurement errors, or confounding variables.
- Assumptions: Discuss any assumptions made in the research process and their implications for the interpretation of results. Assumptions may relate to statistical models, causal inference methods, or theoretical frameworks underlying the study.
- Alternative Explanations: Consider alternative explanations for the observed results and discuss their potential impact on the validity of causal inferences. How robust are the findings to different interpretations or competing hypotheses?
Addressing limitations and assumptions demonstrates transparency and rigor in the research process, allowing readers to critically evaluate the validity and reliability of the findings.
Communicating Findings Clearly
Effectively communicating research findings is essential for disseminating knowledge, informing decision-making, and fostering collaboration and dialogue within the scientific community.
- Clarity and Accessibility: Present findings in a clear, concise, and accessible manner, using plain language and avoiding jargon or technical terminology. Organize information logically and use visual aids (e.g., tables, charts, graphs) to enhance understanding.
- Contextualization: Provide context for the results by summarizing key findings, highlighting their significance, and relating them to existing literature or theoretical frameworks. Discuss the implications of the findings for theory, practice, and future research directions.
- Transparency: Be transparent about the research process, including data collection procedures, analytical methods, and any limitations or uncertainties associated with the findings. Clearly state any conflicts of interest or funding sources that may influence interpretation.
By communicating findings clearly and transparently, researchers can facilitate knowledge exchange, foster trust and credibility, and contribute to evidence-based decision-making.
Causal Research Tips
When conducting causal research, it's essential to approach your study with careful planning, attention to detail, and methodological rigor. Here are some tips to help you navigate the complexities of causal research effectively:
- Define Clear Research Questions: Start by clearly defining your research questions and hypotheses. Articulate the causal relationship you aim to investigate and identify the variables involved.
- Consider Alternative Explanations: Be mindful of potential confounding variables and alternative explanations for the observed relationships. Take steps to control for confounders and address alternative hypotheses in your analysis.
- Prioritize Internal Validity: While external validity is important for generalizability, prioritize internal validity in your study design to ensure that observed effects can be attributed to the manipulation of the independent variable.
- Use Randomization When Possible: If feasible, employ randomization in experimental designs to distribute potential confounders evenly across experimental conditions and enhance the validity of causal inferences.
- Be Transparent About Methods: Provide detailed descriptions of your research methods, including data collection procedures, analytical techniques, and any assumptions or limitations associated with your study.
- Utilize Multiple Methods: Consider using a combination of experimental and observational methods to triangulate findings and strengthen the validity of causal inferences.
- Be Mindful of Sample Size: Ensure that your sample size is adequate to detect meaningful effects and minimize the risk of Type I and Type II errors. Conduct power analyses to determine the sample size needed to achieve sufficient statistical power.
- Validate Measurement Instruments: Validate your measurement instruments to ensure that they are reliable and valid for assessing the variables of interest in your study. Pilot test your instruments if necessary.
- Seek Feedback from Peers: Collaborate with colleagues or seek feedback from peer reviewers to solicit constructive criticism and improve the quality of your research design and analysis.
Conclusion for Causal Research
Mastering causal research empowers researchers to unlock the secrets of cause and effect, shedding light on the intricate relationships between variables in diverse fields. By employing rigorous methods such as experimental designs, causal inference techniques, and careful data analysis, you can uncover causal mechanisms, predict outcomes, and inform evidence-based practices. Through the lens of causal research, complex phenomena become more understandable, and interventions become more effective in addressing societal challenges and driving progress.
In a world where understanding the reasons behind events is paramount, causal research serves as a beacon of clarity and insight. Armed with the knowledge and techniques outlined in this guide, you can navigate the complexities of causality with confidence, advancing scientific knowledge, guiding policy decisions, and ultimately making meaningful contributions to our understanding of the world.
How to Conduct Causal Research in Minutes?
Introducing Appinio, your gateway to lightning-fast causal research. As a real-time market research platform, we're revolutionizing how companies gain consumer insights to drive data-driven decisions. With Appinio, conducting your own market research is not only easy but also thrilling. Experience the excitement of market research with Appinio, where fast, intuitive, and impactful insights are just a click away.
Here's why you'll love Appinio:
- Instant Insights: Say goodbye to waiting days for research results. With our platform, you'll go from questions to insights in minutes, empowering you to make decisions at the speed of business.
- User-Friendly Interface: No need for a research degree here! Our intuitive platform is designed for anyone to use, making complex research tasks simple and accessible.
- Global Reach: Reach your target audience wherever they are. With access to over 90 countries and the ability to define precise target groups from 1200+ characteristics, you'll gather comprehensive data to inform your decisions.
Join the loop 💌
Be the first to hear about new updates, product news, and data insights. We'll send it all straight to your inbox.