What is Nominal Data? Definition, Characteristics, Examples
Appinio Research · 23.02.2024 · 32min read
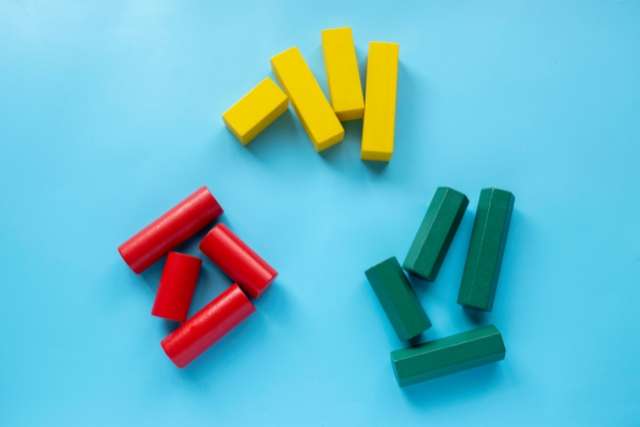
Content
Ever wondered how researchers classify data into different categories? Nominal data holds the key to this classification puzzle. From demographic studies to market research, nominal data helps organize information into distinct groups or labels. But what exactly is nominal data, and why is it essential?
In this guide, we'll explore the basics of nominal data, its characteristics, analysis techniques, and real-world applications. Whether you're a student, researcher, or data enthusiast, understanding nominal data is the first step towards unlocking valuable insights from your datasets.
What is Nominal Data?
Nominal data represents categories or labels that cannot be ordered or ranked in a meaningful way. Unlike numerical data, which can be quantified, nominal data is qualitative in nature, describing attributes rather than quantities.
Importance of Nominal Data
Nominal data holds significant importance across a wide range of disciplines and applications. Some key reasons for its importance include:
- Categorization: Nominal data allows for the classification of observations into distinct categories or groups, facilitating organization and analysis.
- Identification of Patterns: By categorizing data, nominal variables enable the identification of patterns, trends, and associations within datasets.
- Decision Making: Nominal data aids decision-making processes by providing insights into group compositions, preferences, and behaviors.
- Communication: Nominal data helps in effectively communicating information, such as demographic characteristics or group memberships, in a concise and understandable manner.
Nominal Data Characteristics and Properties
Nominal data exhibits specific characteristics and properties that distinguish it from other types of data. These characteristics include:
- Non-Numeric: Nominal data consists of categories or labels that cannot be expressed as numerical values.
- Discreteness: Each category in nominal data is discrete and mutually exclusive, with no overlap between categories.
- No Order: Unlike ordinal data, nominal data categories lack any inherent order or ranking.
- Qualitative: Nominal data describes qualitative attributes or characteristics rather than quantities or measurements.
Understanding these characteristics is essential for appropriately handling and analyzing nominal data in research and analysis.
Nominal Data Applications
Nominal data finds diverse applications across various fields and industries. Some common applications include:
- Demographic Analysis: Nominal data is frequently used in demographic studies to classify individuals based on characteristics such as age, gender, ethnicity, and education level.
- Market Research: In market research, nominal data is employed to segment consumers into distinct groups based on preferences, buying behaviors, or demographic profiles.
- Medical Diagnosis: Nominal data is used in medical diagnosis to classify patients into different diagnostic categories, such as disease types or severity levels.
- Sociological Studies: Sociologists utilize nominal data to examine social phenomena, such as group affiliations, cultural identities, or political affiliations.
These applications highlight the versatility and relevance of nominal data in understanding and interpreting various aspects of the world around us.
Understanding Nominal Variables
Nominal variables play a crucial role in data analysis, providing a framework for categorizing information into distinct groups or labels. Let's delve deeper into the concept of nominal variables, exploring their definition, examples, and how they differ from other data types.
What are Nominal Variables?
Nominal variables are categorical variables that represent distinct categories or labels within a dataset. These categories are used to classify data based on qualitative attributes rather than quantitative measurements. Unlike numerical variables, nominal variables do not have a numerical value associated with them. Instead, they serve as identifiers for different groups or characteristics.
For example, if we are conducting a survey on favorite movie genres and the categories include "Action," "Comedy," "Drama," and "Horror," each genre represents a nominal variable.
Examples of Nominal Variables
Nominal variables are prevalent in various fields and research studies. Here are some common examples:
- Gender: Male, Female, Other
- Marital Status: Single, Married, Divorced, Widowed
- Ethnicity: Caucasian, African American, Hispanic, Asian
- Educational Qualification: High School Diploma, Bachelor's Degree, Master's Degree, PhD
These examples illustrate how nominal variables are used to categorize individuals or entities based on non-quantitative attributes.
Differentiating Nominal Data from Other Data Types
Nominal data differs from other types of data, such as ordinal, interval, and ratio data, based on the level of measurement and the characteristics of the data.
- Ordinal Data: While nominal data categorizes information into distinct groups with no inherent order, ordinal data categorizes information into ordered categories where the relative position or ranking matters. For example, rating scales (e.g., "poor," "fair," "good") represent ordinal data.
- Interval Data: Interval data includes numerical values with equal intervals between them but lacks a true zero point. Unlike nominal data, interval data can be subjected to mathematical operations such as addition and subtraction. However, the zero point is arbitrary, as in the case of temperature measured in Celsius or Fahrenheit.
- Ratio Data: Ratio data possesses the properties of interval data with the addition of a true zero point, where zero represents the absence of the measured quantity. Ratio data allows for meaningful ratios and comparisons between values. Examples include height, weight, and income.
Understanding these distinctions is essential for selecting appropriate statistical methods and interpreting the results accurately in data analysis.
Data Collection Methods for Nominal Data
When it comes to collecting nominal data, researchers have several methods at their disposal, each suited to different research contexts and objectives. Let's explore these methods to understand how nominal data is gathered.
Surveys and Questionnaires
Surveys and questionnaires are popular tools for collecting nominal data, particularly in social sciences, market research, and public opinion studies. These instruments involve presenting respondents with a set of questions or statements, each accompanied by a list of predefined response options. Respondents select the option that best corresponds to their views, preferences, or characteristics.
Surveys and questionnaires offer several advantages for collecting nominal data:
- Scalability: Surveys can be administered to large samples of respondents, making them suitable for studying broad populations or demographic groups.
- Standardization: By using standardized questions and response options, researchers can ensure consistency and comparability across respondents.
- Anonymity: Respondents may feel more comfortable providing honest answers to sensitive questions when their responses are anonymous.
- Efficiency: Surveys can be conducted quickly and cost-effectively, allowing researchers to collect data efficiently.
However, surveys and questionnaires also pose some challenges, such as low response rates, response bias, and the potential to misinterpret questions.
Observational Studies
Observational studies involve systematically observing and recording behaviors, events, or phenomena in their natural settings without intervening or manipulating variables. Researchers collect nominal data by categorizing observed behaviors or characteristics into predefined categories or labels.
Observational studies offer several advantages for collecting nominal data:
- Naturalistic Setting: Observing behaviors in real-world settings allows researchers to capture authentic and spontaneous behavior.
- Flexibility: Observational studies can be adapted to various research contexts and objectives, making them suitable for studying diverse phenomena.
- Richness of Data: Observational data can provide rich, detailed insights into complex behaviors or interactions that may be challenging to capture using other methods.
However, observational studies also have limitations, including potential observer bias, lack of control over extraneous variables, and difficulties in generalizing findings to broader populations.
Experimental Studies
Experimental studies involve manipulating one or more independent variables to observe their effects on a dependent variable. While experimental studies are often associated with quantitative research, nominal data can also be collected within experimental designs by categorizing participants into groups or conditions based on qualitative attributes.
Experimental studies offer several advantages for collecting nominal data:
- Control Over Variables: Experimental designs allow researchers to manipulate variables systematically and control for confounding factors, enhancing the internal validity of the study.
- Causality: By manipulating independent variables and observing their effects on dependent variables, experimental studies can establish causal relationships.
- Replication: Experimental designs can be replicated or repeated to verify findings and ensure the reliability of results.
However, experimental studies also have limitations, including ethical constraints, potential artificiality of laboratory settings, and challenges in generalizing findings to real-world contexts.
Case Studies
Case studies involve in-depth examination and analysis of a single individual, group, organization, or event. Nominal data can be collected within case studies by categorizing attributes, characteristics, or outcomes into predefined categories or labels.
Case studies offer several advantages for collecting nominal data:
- Richness of Data: Case studies provide detailed, in-depth insights into specific cases, allowing researchers to explore complex phenomena in depth.
- Contextual Understanding: By examining individual cases within their broader contexts, case studies can provide a rich, nuanced understanding of real-world phenomena.
- Theory Development: Case studies can generate hypotheses or theories that can be further tested and refined in subsequent research.
However, case studies also have limitations, including potential bias in data collection and analysis, limited generalizability of findings, and challenges in establishing causality.
When considering the myriad of data collection methods for nominal data, it's imperative to weigh the pros and cons of each approach to ensure accurate and insightful results. Whether it's through surveys, observational studies, experimental designs, or case studies, the nuances of data collection can significantly impact the outcomes of your research endeavors.
Harnessing the power of tools like Appinio can streamline this process, allowing you to effortlessly gather real-time consumer insights and make informed decisions with confidence. Dive into the world of data-driven discovery with Appinio and revolutionize the way you conduct research.
Schedule a demo today to experience the seamless integration of consumer insights into your decision-making journey!
Data Representation and Measurement Scales
In data analysis, it's essential to understand different measurement scales and how they influence data representation and analysis. Let's explore the various measurement scales, including nominal, ordinal, interval, and ratio scales, and discuss their significance in data representation.
Nominal Scale
The nominal scale is the simplest form of measurement, where data is categorized into distinct labels or categories with no inherent order or ranking. Each category represents a unique attribute, but there is no quantitative significance to the labels. Nominal data is qualitative in nature and is often used to classify or categorize information.
In nominal scales:
- Categories are mutually exclusive: Each observation belongs to only one category.
- No inherent order: Categories have no natural order or hierarchy.
- Examples: Gender (male, female, other), marital status (single, married, divorced), and types of vehicles (car, truck, motorcycle).
Nominal data is typically represented using frequency counts or percentages within each category. Standard statistical analyses for nominal data include frequency distributions, chi-square tests, and cross-tabulations.
Ordinal Scale
The ordinal scale ranks data into ordered categories or levels, where the relative position or ranking of categories is meaningful. While the categories have a defined sequence, the intervals between them may not be equal or meaningful. Ordinal data retains the qualitative nature of nominal data but adds a degree of order or hierarchy.
In ordinal scales:
- Categories have a meaningful order: Categories are ranked or ordered based on their position.
- Unequal intervals: The differences between categories may not be equal or measurable.
- Examples: Educational attainment (high school diploma, bachelor's degree, master's degree), Likert scale responses (strongly agree, agree, neutral, disagree, strongly disagree).
Interval Scale
The interval scale measures data with equal intervals between consecutive points but lacks a true zero point. While the intervals between values are equal and meaningful, there is no absolute zero point that represents the absence of the measured quantity. Interval data allows for arithmetic operations such as addition and subtraction but not multiplication or division.
In interval scales:
- Equal intervals: The differences between consecutive values are equal and measurable.
- No true zero point: Zero does not represent the absence of the measured quantity.
- Examples: Temperature measured in Celsius or Fahrenheit, dates on the calendar.
Interval data is typically represented using numerical values, and common statistical analyses include mean calculations, standard deviation, and t-tests.
Ratio Scale
The ratio scale is the most informative measurement scale, featuring equal intervals between values and a true zero point where zero represents the absence of the measured quantity. Ratio data allows for meaningful ratios and comparisons between values, as well as all arithmetic operations.
In ratio scales:
- Equal intervals with a true zero point: Zero represents the absence of the measured quantity.
- Meaningful ratios: Ratios between values are meaningful and interpretable.
- Examples: Height, weight, age, income.
Ratio data is represented using numerical values, and common statistical analyses include mean calculations, standard deviation, correlations, and regression analysis.
Comparing Nominal Data with Other Measurement Scales
When comparing nominal data with other measurement scales, it's essential to recognize the qualitative nature of nominal data and its differences from ordinal, interval, and ratio scales.
- Qualitative vs. Quantitative: Nominal data represents qualitative attributes, while ordinal, interval, and ratio data represent quantitative measurements.
- Order and Hierarchy: Nominal data lacks order or hierarchy, while ordinal data has a meaningful order but unequal intervals.
- Arithmetic Operations: Unlike interval and ratio data, nominal and ordinal data cannot be subjected to arithmetic operations such as addition, subtraction, multiplication, or division.
- Statistical Analyses: Different measurement scales require different statistical analyses. Nominal data is often analyzed using non-parametric tests, while interval and ratio data can be analyzed using parametric tests.
Understanding these distinctions is crucial for selecting appropriate data representation techniques, statistical analyses, and interpretation methods in data analysis.
Data Analysis Techniques for Nominal Data
Analyzing nominal data involves various techniques to summarize, visualize, and interpret categorical information. Let's explore these techniques and understand how they contribute to gaining insights from nominal data.
Frequency Distribution
Frequency distribution is a fundamental technique for analyzing nominal data, providing a summary of the number of occurrences of each category within a dataset. It helps identify patterns, trends, and distributions within the data by counting the frequency of each category.
To Create a Frequency Distribution:
- Identify Categories: Determine the distinct categories or labels within the nominal dataset.
- Count Frequencies: Count the number of observations belonging to each category.
- Tabulate Data: Organize the frequencies into a table format, listing each category along with its corresponding frequency count.
- Visualize Data: Visualize the frequency distribution using bar charts or pie charts to enhance understanding and interpretation.
Frequency distributions provide valuable insights into the distribution of categorical variables, allowing researchers to identify dominant categories, outliers, and patterns of interest.
Mode
The mode is a measure of central tendency that represents the most frequently occurring category or value within a dataset. For nominal data, the mode is simply the category with the highest frequency count.
To Calculate the Mode:
- Identify Categories: Determine the distinct categories within the dataset.
- Count Frequencies: Calculate the frequency count for each category.
- Find the Mode: Identify the category with the highest frequency count.
The mode is particularly useful for identifying the most common or prevalent category within a dataset. It provides a simple and intuitive summary of the central tendency of nominal data.
Chi-square Test
The chi-square test is a statistical test used to determine whether there is a significant association between two categorical variables. It compares the observed frequencies of categories with the expected frequencies under the assumption of independence between the variables.
To Conduct a Chi-square Test:
- Formulate Hypotheses: Define the null hypothesis (no association) and alternative hypothesis (association) based on the research question.
- Calculate Expected Frequencies: Calculate the expected frequencies for each category under the assumption of independence.
- Compute Test Statistic: Calculate the chi-square statistic using the formula:
χ^2 = ∑((Oi - Ei)^2 / Ei)
Where Oi is the observed frequency, and Ei is the expected frequency for each category. - Assess Significance: Compare the calculated chi-square statistic with the critical value from the chi-square distribution to determine statistical significance.
The chi-square test is widely used in various fields, including social sciences, market research, and epidemiology, to assess relationships between categorical variables.
Cross-tabulation
Cross-tabulation, also known as contingency table analysis, is a technique for examining the relationship between two or more categorical variables by organizing data into a table format. It allows researchers to compare the distribution of categories across different groups or conditions.
To Conduct Cross-tabulation:
- Identify Variables: Select the categorical variables of interest for cross-tabulation.
- Create Contingency Table: Construct a contingency table with rows representing one variable and columns representing another variable.
- Calculate Frequencies: Count the frequencies of observations for each combination of categories.
- Interpret Results: Analyze the patterns and associations observed in the contingency table to draw conclusions about the relationship between variables.
Cross-tabulation is a powerful tool for exploring interactions and dependencies between categorical variables, providing valuable insights into the underlying structure of the data.
Bar Charts and Pie Charts
Bar charts and pie charts are graphical representations of nominal data, visually displaying the distribution of categories within a dataset. These visualizations help researchers and stakeholders understand the relative frequencies of different categories and identify patterns or trends.
- Bar Charts: Bar charts represent categorical data using rectangular bars of varying lengths, with each bar corresponding to a category and its height proportional to the frequency count.
- Pie Charts: Pie charts display categorical data as a circular diagram divided into slices, with each slice representing a category and its size proportional to the frequency count.
Bar charts and pie charts are effective tools for communicating findings and presenting insights in a visually appealing format. They are widely used in reports, presentations, and publications to convey key messages derived from nominal data analysis.
Interpretation of Nominal Data Analysis
After analyzing nominal data, it's crucial to interpret the results accurately to draw meaningful conclusions and insights. Let's explore the interpretation of nominal data analysis in various contexts, including frequency distributions, cross-tabulations, chi-square test results, and effective communication of findings.
Drawing Conclusions from Frequency Distributions
Frequency distributions provide a summary of the number of occurrences of each category within a dataset. To draw conclusions from frequency distributions:
- Identify Dominant Categories: Determine which categories have the highest frequencies, indicating the most prevalent attributes within the dataset.
- Identify Outliers: Look for categories with unusually high or low frequencies compared to others, which may indicate unique or rare attributes.
- Identify Patterns: Analyze the distribution of categories to identify any patterns or trends, such as clustering or dispersion of data.
- Compare Subgroups: If applicable, compare frequency distributions across different subgroups or conditions to identify differences or similarities.
Interpreting frequency distributions allows researchers to gain insights into the distribution and prevalence of different attributes within the dataset, informing further analysis and decision-making.
Analyzing Patterns in Cross-tabulations
Cross-tabulations provide a means to examine the relationship between two or more categorical variables by organizing data into a table format. To analyze patterns in cross-tabulations:
- Examine Cell Counts: Review the frequencies of observations in each cell of the contingency table to identify patterns or associations.
- Calculate Percentages: Calculate row percentages, column percentages, or total percentages to compare the distribution of categories across different variables.
- Test for Independence: Use statistical tests, such as the chi-square test, to determine whether there is a significant association between variables.
Analyzing patterns in cross-tabulations helps identify relationships and dependencies between categorical variables, providing insights into the underlying structure of the data.
Interpreting Chi-square Test Results
The chi-square test is a statistical test used to determine whether there is a significant association between two categorical variables. To interpret chi-square test results:
- Compare Observed and Expected Frequencies: Review the calculated chi-square statistic and compare it to the critical value from the chi-square distribution.
- Assess Significance: Determine whether the chi-square statistic is statistically significant at a predetermined level of significance (e.g., p < 0.05).
- Interpret Effect Size: Consider the effect size measures, such as Cramer's V or Phi coefficient, to assess the strength of the association between variables.
- Examine Residuals: Analyze standardized residuals to identify specific cells contributing to the observed association.
Interpreting chi-square test results helps determine whether there is evidence of a significant association between categorical variables and provides insights into the nature and strength of the relationship.
Communicating Findings Effectively
Effective communication of findings is essential for conveying insights derived from nominal data analysis to stakeholders and decision-makers. To communicate findings effectively:
- Use Clear and Concise Language: Present findings in plain language, avoiding jargon or technical terms that may be unfamiliar to the audience.
- Use Visualizations: Utilize graphical representations, such as bar charts, pie charts, or tables, to visually illustrate key findings and trends.
- Provide Context: Offer context for the findings by explaining the significance of the results and their implications for decision-making or further research.
- Tailor Messaging to the Audience: Consider the needs and preferences of the audience when communicating findings, adapting the message to resonate with their interests and priorities.
Effective communication of findings ensures that insights derived from nominal data analysis are understood and utilized to inform decision-making and drive action.
Nominal Data Examples
Understanding nominal data is essential for various fields and industries. Let's delve into some detailed examples to grasp how nominal data manifests in different contexts.
Demographic Data
Demographic studies rely heavily on nominal data to classify individuals based on various attributes:
- Gender: Male, Female, Non-binary
- Ethnicity: Caucasian, African American, Hispanic, Asian
- Marital Status: Single, Married, Divorced, Widowed
- Education Level: High School Diploma, Bachelor's Degree, Master's Degree, PhD
Product Categories
In retail and market research, products are categorized into distinct groups:
- Apparel: Tops, Bottoms, Dresses, Accessories
- Food: Dairy, Produce, Meat, Frozen Foods
- Electronics: Smartphones, Laptops, Televisions, Headphones
Survey Responses
Survey data often involves nominal variables to categorize responses:
- Preferred Communication Method: Email, Phone, In-person
- Likert Scale Responses: Strongly Agree, Agree, Neutral, Disagree, Strongly Disagree
- Political Affiliation: Republican, Democrat, Independent, Other
Medical Diagnosis
Medical diagnoses are classified using nominal data to distinguish different conditions:
- Disease Status: Infected, Non-infected
- Cancer Subtypes: Breast Cancer, Lung Cancer, Prostate Cancer
- Severity Levels: Mild, Moderate, Severe
Geographic Regions
Geographic data is categorized into regions and zones:
- Continents: Africa, Asia, Europe, North America, South America
- Climate Zones: Tropical, Temperate, Polar
- Administrative Units: Countries, States, Provinces, Cities
Examining these examples gives you a deeper understanding of how nominal data is applied across various domains. Whether it's analyzing demographics, market segments, survey responses, medical conditions, or geographical regions, nominal data provides a versatile framework for classification and interpretation.
Nominal Data Challenges and Considerations
Working with nominal data presents several challenges and considerations that researchers must address to ensure accurate analysis and interpretation. These challenges include:
- Data Quality and Accuracy: Ensuring the quality and accuracy of nominal data is essential for reliable analysis. Common issues include missing values, misclassification, and data entry errors. Implementing data validation checks and cleaning procedures is vital to minimize errors and improve data quality.
- Handling Missing Values: Missing values can introduce bias and affect the validity of analysis results. Researchers must develop strategies for handling missing data, such as imputation techniques, deletion of incomplete cases, or sensitivity analysis to assess the impact of missingness on results.
- Dealing with Large Datasets: Large datasets present challenges in terms of processing power, storage, and analysis techniques. Researchers must develop strategies for efficiently managing and analyzing large volumes of nominal data, such as data sampling, parallel computing, or distributed computing frameworks.
- Addressing Bias and Confounding Factors: Bias and confounding factors can distort analysis results and lead to erroneous conclusions. Researchers must be vigilant in identifying and controlling for potential sources of bias, such as selection bias, measurement bias, or confounding variables. Strategies include randomization, blinding, and statistical adjustment techniques.
Best Practices for Handling Nominal Data
To ensure effective handling and analysis of nominal data, researchers should adhere to best practices throughout the data lifecycle. These best practices include:
- Data Cleaning and Preprocessing: Thoroughly clean and preprocess nominal data before analysis to address missing values, outliers, and inconsistencies. This may involve data validation, transformation, and normalization techniques to improve data quality and consistency.
- Choosing Appropriate Analysis Techniques: Select analysis techniques that are suitable for nominal data, such as frequency distributions, chi-square tests, or cross-tabulations. Consider the research question, data characteristics, and assumptions of the analysis techniques when choosing appropriate methods.
- Ensuring Data Privacy and Security: Protect the privacy and confidentiality of nominal data by implementing appropriate security measures, such as encryption, access controls, and anonymization techniques. Comply with data protection regulations and ethical guidelines to safeguard sensitive information.
- Documenting Data and Analysis Procedures: Maintain comprehensive documentation of nominal data and analysis procedures to ensure transparency, reproducibility, and auditability. Document data sources, variables, coding schemes, and analysis techniques to facilitate replication and validation of results.
Adhering to these best practices helps ensure the reliability, validity, and reproducibility of nominal data analysis, leading to more robust and trustworthy research outcomes.
Conclusion for Nominal Data
Nominal data plays a vital role in data analysis across various fields and industries. By categorizing information into distinct labels or categories, nominal data enables researchers to organize, analyze, and interpret complex datasets effectively. From demographic studies to market research and medical diagnosis, nominal data provides valuable insights into group characteristics, preferences, and behaviors. Understanding the basics of nominal data, including its definition, characteristics, and analysis techniques, empowers individuals to make informed decisions and draw meaningful conclusions from their data.
In today's data-driven world, the importance of nominal data cannot be overstated. Whether you're conducting research, making business decisions, or simply exploring patterns in your data, nominal data serves as a fundamental building block for analysis and interpretation. By mastering the concepts and techniques outlined in this guide, you'll be equipped with the knowledge and skills needed to harness the power of nominal data and unlock actionable insights that drive success in your endeavors.
How to Collect Nominal Data in Minutes?
Discover the power of real-time consumer insights with Appinio, your go-to platform for collecting nominal data effortlessly. As a real-time market research platform, Appinio empowers businesses to make informed, data-driven decisions swiftly. With Appinio, conducting your own market research becomes a breeze, allowing you to gain valuable insights in minutes.
Here's why you should choose Appinio:
- From questions to insights in minutes: Appinio streamlines the research process, delivering actionable insights in record time.
- Intuitive platform for everyone: No need for a research PhD; our user-friendly interface ensures anyone can navigate and utilize the platform effectively.
- Extensive reach and targeting options: With access to over 90 countries and the ability to define target groups using 1200+ characteristics, Appinio ensures that you reach the right audience for your nominal data collection needs.
Get facts and figures ðŸ§
Want to see more data insights? Our free reports are just the right thing for you!