Interval Scale: Definition, Characteristics, Examples
Appinio Research · 07.05.2024 · 30min read
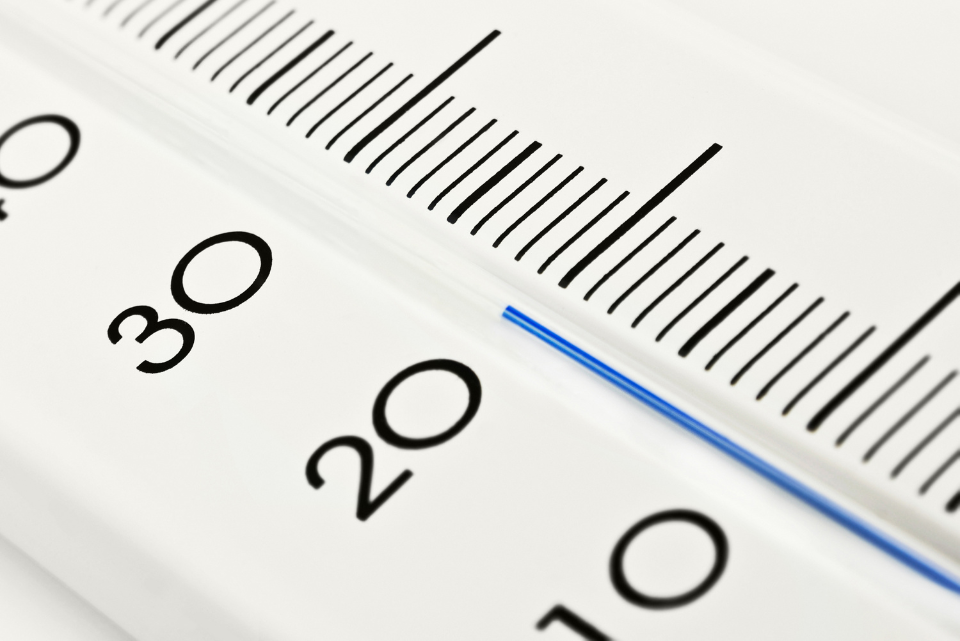
Content
Have you ever wondered how we measure things like temperature, IQ, or time intervals? Understanding how we quantify these attributes is essential for making sense of the world around us. One important concept in measurement is the interval scale, which provides a structured framework for comparing variables based on their magnitude. Interval scale measurements offer precise numerical comparisons while maintaining equal intervals between data points. However, unlike scales with a true zero point, such as the ratio scale, interval scale measurements have an arbitrary zero point, which serves as a reference rather than an absolute absence of the measured attribute.
In this guide, we'll explore the intricacies of interval scales, their practical applications across various fields, and the best practices for collecting, analyzing, and interpreting interval scale data. Whether you're a researcher, educator, or market analyst, understanding interval scales is fundamental for making informed decisions and deriving meaningful insights from quantitative data.
What is an Interval Scale?
An interval scale is a type of measurement scale that ranks variables based on their magnitude. The difference between any two points on the scale is meaningful and consistent. Unlike nominal and ordinal scales, which categorize variables without implying numerical relationships, interval scales incorporate equal intervals between measurements, allowing for quantitative comparisons.
The basic concept of an interval scale revolves around maintaining equal intervals between measurements while preserving the order of variables. This means that the difference between any two adjacent points on the scale remains constant and meaningful, regardless of the specific units or attributes being measured.
Importance of Interval Scales in Data Measurement
Interval scales play a crucial role in quantitative research and data measurement across various fields. Its importance stems from several key factors that distinguish it from other measurement scales:
- Quantitative Comparisons: Interval scales enable researchers to make precise numerical comparisons between variables, facilitating rigorous analysis and interpretation of data. By quantifying differences and relationships with accuracy, interval scales enhance the reliability and validity of research findings.
- Statistical Analysis: Interval scale data lends itself to a wide range of statistical techniques, including descriptive and inferential statistics. Researchers can apply statistical tests, regression models, and hypothesis testing procedures to interval scale data to uncover patterns, test hypotheses, and make informed decisions.
- Standardization and Consistency: Using equal intervals in interval scale measurements ensures standardization and consistency in data representation. This allows for standardized comparisons across different measurement units and ensures that data collected using interval scale measures are comparable and interpretable.
- Precision and Detail: The interval scale provides a level of accuracy and detail that may not be achievable with other measurement scales, such as nominal or ordinal scales. By incorporating numerical values and equal intervals, the interval scale captures finer distinctions and nuances in the measured attributes, enhancing the richness and depth of data analysis.
Interval Scale Characteristics
Understanding the main characteristics of the interval scale is essential for recognizing its distinctive properties and applications. Here are some key characteristics that define an interval scale:
- Equal Intervals: The interval scale maintains consistent intervals between measurements, ensuring uniformity and precision in data representation. Each unit on the scale represents an equal increment of the measured attribute, facilitating accurate comparisons and analysis.
- Arbitrary Zero Point: The interval scale lacks a true zero point and features an arbitrary reference point instead. While this zero point serves as a reference for numerical comparisons, it does not imply an absence of the measured attribute. This characteristic distinguishes the interval scale from the ratio scale, where zero represents a true absence.
- Numerical Rankings: Variables measured on an interval scale can be ranked based on their magnitude, allowing for quantitative comparisons and statistical analysis. The interval scale preserves the order of variables while incorporating numerical values, enabling researchers to assess relationships and trends systematically.
By understanding these key characteristics, researchers can effectively apply interval scale in data measurement, analysis, and interpretation, enhancing the quality and reliability of research outcomes.
Understanding Interval Scales
Interval scale is a fundamental concept in data measurement, offering valuable insights into the quantitative assessment of variables. To fully grasp its significance, let's explore its relationship with other measurement scales, examine real-life examples, and understand its properties and limitations.
Relationship with Other Measurement Scales
Interval scale occupies a unique position among measurement scales, bridging the gap between ordinal and ratio scales. While ordinal scales merely rank variables without specifying the magnitude of differences between them, interval scales introduce the concept of equal intervals, enabling numerical comparisons. However, unlike ratio scales, interval scales lack a true zero point, restricting certain mathematical operations.
To illustrate, consider a scenario where you're measuring temperature using the Fahrenheit scale. Each degree represents an equal interval, allowing for precise comparisons between temperatures. However, the zero point on the Fahrenheit scale (-32°F) doesn't signify a complete absence of temperature but rather an arbitrary reference point. This distinction highlights the unique characteristics of interval scale data and its implications for statistical analysis.
Interval Scale Examples
Interval scale measurements are pervasive in various domains, from scientific research to everyday observations. Let's explore some common examples of interval scale measurements in real life:
- Temperature: Whether using the Celsius, Fahrenheit, or Kelvin scale, temperature measurements exemplify interval scale data. Each degree represents an equal interval, enabling precise comparisons between temperatures.
- IQ Scores: Intelligence quotient (IQ) scores obtained from standardized tests provide interval scale measurements of cognitive abilities. While IQ scores rank individuals based on their relative intelligence levels, the intervals between scores remain consistent, facilitating meaningful comparisons.
- Time Intervals: Time measurements, such as seconds, minutes, and hours, also adhere to the principles of interval scale. While time intervals maintain equal increments, the zero point (e.g., the start of a stopwatch) serves as an arbitrary reference rather than an absolute absence of time.
Interval Scale Properties and Limitations
Interval scales possess distinct properties and limitations that shape their utility in data measurement and analysis. Let's delve into these aspects to comprehend the nuances of interval scales more comprehensively.
Interval Scale Properties
- Equal Intervals: The interval scale maintains consistent intervals between measurements, facilitating precise numerical comparisons.
- Numerical Rankings: Variables measured on an interval scale can be ranked based on their magnitude, providing a structured framework for quantitative analysis.
- Flexibility: Interval scale offers greater flexibility than ordinal scales by incorporating numerical values for comparisons.
Interval Scale Limitations
- Arbitrary Zero Point: The absence of a true zero point restricts certain mathematical operations, such as division by zero, and complicates interpretation.
- Limited Scale Range: Interval scale measurements may have a finite range, making it challenging to capture extreme values accurately.
Understanding these properties and limitations is crucial for effectively utilizing interval scale data in research, decision-making, and problem-solving scenarios.
Interval Scale Features
Understanding the key features of interval scale is essential for leveraging its benefits effectively. Let's explore the significance of the zero point, the concept of equal intervals, and the diverse applications of interval scale across various fields.
Zero Point and its Significance
The zero point in interval scale measurements serves as a crucial reference point, albeit with distinct characteristics compared to the zero point in ratio scale measurements. Unlike in ratio scales, where zero denotes an absolute absence of the measured attribute, the zero point in interval scales is arbitrary, representing a reference rather than a true absence.
To illustrate, consider measuring temperature using the Kelvin scale. While absolute zero (-273.15°C) signifies the complete absence of molecular motion, the zero point on the Kelvin scale (0 K) serves as a reference point without implying the absence of temperature. This distinction is fundamental in understanding interval scale measurements and their implications for data interpretation.
In practical terms, the arbitrary zero point allows for meaningful comparisons between measurements but imposes limitations on certain mathematical operations. For instance, while addition and subtraction are valid operations on interval scale data, division by zero is undefined due to the absence of a true zero point.
Formula:
X(interval​) = X(raw)​ − X(min)​
Where:
- X(interval)​ is the interval scale measurement.
- X(raw​) is the raw score.
- X(min)​ is the minimum score in the dataset.
Equal Intervals and Measurement Units
One of the defining characteristics of interval scale is the maintenance of equal intervals between measurements. This feature ensures uniformity in data representation and facilitates precise comparisons between variables.
When measuring variables on an interval scale, each unit represents a consistent interval of the attribute being measured. For example, in measuring time intervals using minutes, each minute represents an equal duration, allowing for accurate comparisons between different time spans.
Moreover, interval scale measurements are not limited to specific units but can encompass a wide range of measurement units depending on the attribute being assessed. Whether measuring temperature in Celsius, Fahrenheit, or Kelvin, the concept of equal intervals remains consistent, enabling standardized comparisons across different scales.
Interval Scale Applications
Interval scale finds widespread application across diverse fields, owing to its versatility and precision in data measurement. Let's explore some key domains where interval scale plays a pivotal role:
- Scientific Research: In scientific studies, interval scale measurements are indispensable for quantifying variables such as temperature, pH levels, and reaction times. These measurements form the basis for hypothesis testing, data modeling, and scientific discoveries.
- Market Research and Consumer Behavior Analysis: Market researchers utilize interval scale data to assess consumer preferences, satisfaction levels, and purchasing behavior. By quantifying customer perceptions and attitudes, businesses can tailor marketing strategies and product offerings effectively.
- Education and Testing: In educational assessments, interval scale measurements inform evaluations of student performance and academic achievement. Standardized tests, IQ assessments, and proficiency exams provide interval scale data that guides educational policies, curriculum development, and instructional practices.
By recognizing the diverse applications of interval scale across these fields, you gain a deeper appreciation for its role in facilitating data-driven decision-making and advancing knowledge in various domains.
Interval Scale Data Collection and Analysis Methods
To harness the power of interval scale data effectively, it's crucial to employ robust methods for both data collection and data analysis. We'll delve into various techniques for collecting interval scale data, statistical approaches for analysis, and strategies for interpreting results accurately.
Data Collection Techniques
Collecting interval scale data requires careful consideration of the research context and the attributes being measured. Here are some commonly used techniques:
- Surveys and Questionnaires: Surveys and questionnaires are versatile tools for collecting interval scale data on subjective attributes such as attitudes, preferences, and opinions. Researchers can design structured surveys with Likert scales or numerical rating scales to quantify respondents' perceptions accurately.
Example: In a customer satisfaction survey, respondents rate their satisfaction levels on a scale from 1 to 5, with 1 indicating "very dissatisfied" and 5 indicating "very satisfied." - Observational Studies: Observational studies involve directly observing phenomena or behaviors to collect interval scale data. Researchers can record quantitative observations, such as reaction times, response frequencies, or event durations, to gather empirical data for analysis.
Example: A researcher observes and records the time taken by participants to complete a task in a usability study, yielding interval scale data on task efficiency. - Experimental Designs: Experimental designs allow researchers to manipulate variables under controlled conditions to collect interval scale data on experimental outcomes. Researchers can assess the effects of interventions or treatments by randomly assigning participants to treatment groups and measuring outcomes quantitatively.
Example: A clinical trial investigates the efficacy of a new drug by measuring changes in patients' blood pressure levels before and after treatment, providing interval scale data on treatment effects.
Having the right tools for data collection is paramount to unlocking the potential of interval scale data. Appinio offers a seamless solution for gathering insights, empowering researchers to streamline the process and focus on extracting valuable information. With Appinio's intuitive platform and global reach, researchers can effortlessly design surveys, target specific demographics, and receive actionable feedback in record time.
Ready to take your research to the next level? Book a demo with Appinio today and discover the power of real-time consumer insights!
Statistical Analysis Approaches
Once interval scale data is collected, various statistical techniques can be applied to analyze the data and derive meaningful insights:
- Descriptive Statistics: Descriptive statistics summarize and describe the characteristics of interval scale data, providing insights into central tendency, dispersion, and distribution. Standard measures include mean, median, mode, range, and standard deviation.
Example: Calculating the mean temperature across multiple days provides a summary measure of the central tendency of temperature data. - Inferential Statistics: Inferential statistics enable researchers to make inferences and draw conclusions about populations based on sample data. Techniques such as hypothesis testing, regression analysis, and analysis of variance (ANOVA) are commonly used to test relationships, compare groups, and evaluate hypotheses.
Example: Conducting a t-test to compare the mean scores of two groups on an interval scale variable determines whether the difference between the groups is statistically significant.
Interpretation of Results
Interpreting interval scale data requires careful consideration of the context, research objectives, and statistical findings. Some strategies for interpreting results include:
- Contextual Understanding: Interpret results within the context of the research question or hypothesis being investigated. Consider how the findings contribute to existing knowledge or address the research objectives.
- Statistical Significance: Assess the statistical significance of findings using appropriate tests and confidence intervals. Determine whether observed differences or relationships are likely to be meaningful or due to chance.
- Practical Significance: Evaluate the practical significance or real-world implications of results. Consider whether observed effects are substantial enough to warrant practical action or decision-making.
- Limitations and Caveats: Acknowledge any limitations or caveats associated with the data collection methods, statistical analyses, or interpretation of results. Consider alternative explanations or factors that may influence the findings.
By employing rigorous data collection techniques, applying appropriate statistical analyses, and critically interpreting the results, researchers can derive meaningful insights from interval scale data and make informed decisions based on empirical evidence.
Interval Scale Advantages and Disadvantages
Understanding the advantages and disadvantages of interval scales is crucial for informed decision-making and effective utilization of quantitative data. Let's explore the benefits of interval scales, their limitations and challenges, and how they compare to other measurement scales.
Advantages of Interval Scale
Interval scale offers several advantages that make it a valuable tool in data measurement and analysis:
- Quantitative Comparisons: The interval scale allows for precise numerical comparisons between variables, facilitating rigorous data analysis and interpretation. Researchers can quantify differences and relationships with greater accuracy compared to ordinal or nominal scales.
- Statistical Analysis: The numerical nature of interval scale data enables researchers to apply a wide range of statistical techniques for hypothesis testing, data modeling, and inference. Descriptive and inferential statistics can be effectively employed to derive meaningful insights from interval scale measurements.
- Flexibility: Interval scale provides greater flexibility than ordinal scales by incorporating numerical values for comparisons. This flexibility allows researchers to capture a wide range of variation in measured attributes, enabling comprehensive analysis and interpretation of data.
- Equal Intervals: Maintaining equal intervals between measurements ensures uniformity in data representation and facilitates standardized comparisons across different measurement units. This feature enhances the reliability and validity of interval scale measurements, enabling consistent and reproducible results.
Interval Scale Limitations and Challenges
Despite its advantages, the interval scale also has several limitations and challenges that researchers should be aware of:
- Arbitrary Zero Point: The absence of a true zero point in interval scale measurements restricts certain mathematical operations and complicates interpretation. Division by zero is undefined, and ratios between measurements are not meaningful due to the arbitrary nature of the zero point.
- Limited Scale Range: Interval scale measurements may have a finite range, making it challenging to capture extreme values accurately. Researchers should be cautious when interpreting interval scale data near the boundaries of the scale range to avoid extrapolation beyond the observed data.
- Subjectivity: Interval scale data may be subject to subjective interpretation, particularly in self-reported measures or assessments of abstract constructs. When interpreting interval scale data, researchers should consider potential biases and variations in respondents' perceptions.
- Complexity of Analysis: Analyzing interval scale data may require advanced statistical techniques and computational methods, particularly when dealing with multiple variables or complex relationships. Researchers should have the expertise and resources to handle and interpret interval scale data effectively.
Interval Scale vs. Other Measurement Scales
Comparing interval scale with nominal, ordinal, and ratio scales highlights its unique characteristics and applications:
- Nominal Scale: The interval scale offers greater precision and flexibility than the nominal scale, allowing for numerical comparisons and statistical analysis of data. Unlike the nominal scale, which merely categorizes variables without specifying magnitude or order, the interval scale provides a structured framework for quantitative analysis.
- Ordinal Scale: An interval scale introduces the concept of equal intervals, distinguishing it from an ordinal scale, where rankings are meaningful, but intervals between categories may not be uniform. While an ordinal scale provides ordinal relationships between variables, an interval scale enables precise numerical comparisons and statistical inference.
- Ratio Scale: A ratio scale features a true zero point, allowing for meaningful ratios and absolute comparisons between measurements. In contrast, an interval scale's arbitrary zero point limits certain mathematical operations and interpretive capabilities, particularly when dealing with ratios or proportions.
Understanding the distinctions between interval scales and other measurement scales is essential for selecting appropriate measurement methods, designing research studies, and interpreting data effectively. By recognizing the advantages and limitations of interval scales, researchers can make informed decisions and derive meaningful insights from quantitative data.
Interval Scale Applications
Interval scale finds extensive practical applications across various domains, from scientific research to market analysis and education. Let's explore how interval scales are utilized in these fields and their impact on decision-making and knowledge advancement.
Scientific Research
In scientific research, interval scale measurements serve as the foundation for empirical investigations and data-driven discoveries. Here are some common applications of interval scale in scientific research:
- Quantitative Analysis: Interval scale data enables researchers to quantify variables such as temperature, time, and physical properties with precision. By collecting interval scale measurements, scientists can analyze trends, detect patterns, and draw conclusions based on empirical evidence.
- Experimental Design: Interval scale measurements are essential for designing controlled experiments and assessing the effects of interventions or treatments. Whether studying the efficacy of pharmaceutical drugs, evaluating environmental factors, or investigating biological processes, interval scale data provides quantitative insights into experimental outcomes.
- Statistical Modeling: Interval scale data facilitates statistical modeling and hypothesis testing in scientific research. Researchers can apply advanced statistical techniques, such as regression analysis, analysis of variance (ANOVA), and multivariate analysis, to explore relationships between variables, predict outcomes, and infer population parameters from sample data.
- Data Visualization: Interval scale data visualization techniques, such as histograms, scatter plots, and line graphs, enhance the interpretation and communication of research findings. Visual representations of interval scale data enable researchers to identify trends, outliers, and relationships intuitively, facilitating data-driven decision-making and knowledge dissemination.
Market Research and Consumer Behavior Analysis
In market research and consumer behavior analysis, interval scale measurements play a crucial role in understanding consumer preferences, attitudes, and purchasing behavior. Here's how interval scales are applied in these fields:
- Survey Research: Market researchers use interval scale data obtained from surveys and questionnaires to assess consumer satisfaction, brand perceptions, and product preferences. Researchers can quantify consumer sentiments and identify market trends by collecting interval scale measurements on Likert scales or numerical rating scales.
- Segmentation Analysis: Interval scale data enables segmentation analysis, where consumers are grouped based on shared characteristics or preferences. By clustering consumers into segments based on interval scale variables such as income, age, or purchasing frequency, marketers can tailor marketing strategies and product offerings to target specific market segments.
- Conjoint Analysis: Conjoint analysis is a technique used in market research to assess consumer preferences for different product attributes or features. Researchers can quantify consumer trade-offs and optimize product design and pricing strategies by presenting consumers with hypothetical product profiles and collecting interval scale ratings on attribute importance or preference.
- Predictive Modeling: Interval scale data is utilized in predictive modeling to forecast consumer behavior and market trends. By applying statistical techniques such as regression analysis and machine learning algorithms to interval scale data, marketers can develop predictive models that anticipate consumer preferences, demand patterns, and purchasing behavior.
Education and Testing
In education and testing, interval scale measurements provide valuable insights into student performance, academic achievement, and learning outcomes. Here's how the interval scale is applied in educational assessments and testing:
- Standardized Testing: Interval scale measurements obtained from standardized tests, such as achievement tests, IQ assessments, and proficiency exams, enable educators to assess student knowledge, skills, and academic proficiency objectively. Educators can evaluate student performance relative to established benchmarks and norms by administering standardized tests with interval scale scoring.
- Assessment of Learning Outcomes: Interval scale measurements are used to assess learning outcomes and educational effectiveness. By measuring student progress and achievement using interval scale assessments, educators can identify areas for improvement, tailor instructional strategies, and monitor student growth over time.
- Program Evaluation: Interval scale data is utilized in program evaluation to assess the effectiveness of educational interventions, curriculum reforms, and instructional initiatives. By collecting interval scale measurements on student outcomes, such as test scores, grades, and graduation rates, educators and policymakers can evaluate the impact of educational programs and inform evidence-based decision-making.
- Data-Driven Instruction: Interval scale data analysis informs data-driven instructional practices, where educators use empirical evidence to guide instructional decisions and interventions. By analyzing interval scale data on student performance and learning outcomes, educators can identify instructional needs, differentiate instruction, and provide targeted support to students.
By recognizing the practical applications of interval scale in scientific research, market analysis, consumer behavior analysis, education, and testing, stakeholders can leverage interval scale data to make informed decisions, drive innovation, and advance knowledge in their respective fields.
Best Practices for Utilizing Interval Scales
Utilizing interval scales requires adherence to best practices in data collection, analysis, and interpretation. To maximize the utility of interval scale data:
- Standardized Measurement Protocols: Adopt standardized measurement protocols to ensure consistency and accuracy in interval scale data collection. Establish clear procedures for administering surveys, conducting observations, or implementing experimental designs to minimize measurement error and enhance data quality.
- Calibration of Instruments: Regularly calibrate measuring instruments to maintain accuracy and reliability in interval scale measurements. Calibration ensures that measurement devices are properly calibrated and calibrated according to industry standards, reducing variability and enhancing the validity of interval scale data.
- Random Sampling Techniques: Employ random sampling techniques to reduce sampling bias and ensure the representativeness of study populations. Random sampling helps mitigate selection bias and ensures that interval scale data accurately reflects the target population's characteristics, enhancing the generalizability of research findings.
- Error Analysis and Validation: Conduct thorough error analysis to identify and mitigate sources of measurement error in interval scale data. Validate measurement instruments and procedures through pilot testing, reliability testing, and validity assessment to ensure the robustness of interval scale measurements.
- Data Cleaning and Preprocessing: Prioritize data cleaning and preprocessing to identify and correct errors, outliers, or missing values in interval scale data. Implement data validation checks, outlier detection algorithms, and imputation techniques to enhance the integrity and completeness of interval scale datasets before analysis.
- Transparent Reporting and Documentation: Document and report interval scale data collection methods, analysis procedures, and results transparently to facilitate reproducibility and transparency in research. Provide detailed descriptions of measurement instruments, sampling procedures, and statistical analyses to enable peer review and scrutiny of research findings.
- Ethical Considerations: Adhere to ethical principles and guidelines in the collection, analysis, and reporting of interval scale data. Ensure informed consent, confidentiality, and privacy protection for research participants, and adhere to institutional review board (IRB) protocols and ethical standards to uphold the integrity and trustworthiness of interval scale research.
- Continuous Quality Improvement: Continuously monitor and improve interval scale data collection and analysis processes to enhance data quality and validity. Solicit feedback from stakeholders, conduct post-hoc evaluations, and implement quality improvement initiatives to address emerging challenges and optimize research practices.
By following these best practices for utilizing interval scales, researchers can ensure the reliability, validity, and integrity of interval scale data, enabling robust analysis, interpretation, and dissemination of research findings.
Conclusion for Interval Scales
The interval scale is a versatile and powerful tool for quantitative measurement and analysis. By providing a structured framework for comparing variables with equal intervals, interval scales enable researchers to make precise numerical comparisons and derive meaningful insights from data. From scientific research to market analysis and education, interval scale finds wide-ranging applications, shaping decision-making and driving innovation across diverse fields. By adhering to best practices and understanding the key characteristics of interval scales, researchers can harness their full potential to advance knowledge, inform policies, and solve complex problems.
In today's data-driven world, interval scale is a cornerstone of quantitative research and analysis. Its unique characteristics, including equal intervals and numerical rankings, make it indispensable for making sense of complex phenomena and making informed decisions based on empirical evidence. Whether you're conducting scientific experiments, analyzing consumer behavior, or evaluating student performance, interval scales provide a reliable and robust framework for quantifying variables and uncovering meaningful patterns and trends. As you apply the principles of interval scale in your own work, remember to consider its limitations and challenges and to continually strive for improvement and innovation in your research practices.
How to Collect Data in Minutes?
Introducing Appinio, the real-time market research platform that revolutionizes how companies gather consumer insights. With Appinio, you can easily collect data for interval scales in minutes, empowering you to make better data-driven decisions for your business.
Here's why you should choose Appinio:
- From questions to insights in minutes: Appinio streamlines the entire research process, delivering actionable insights at lightning speed. Say goodbye to lengthy waiting times and hello to rapid decision-making.
- Intuitive platform for everyone: No PhD in research? No problem. Appinio's user-friendly interface makes it easy for anyone to design and launch surveys without the need for specialized knowledge.
- Global reach with targeted precision: With over 90 countries covered and 1200+ characteristics to define your target group, Appinio ensures that you reach the right audience for your research, no matter where they are.
Get facts and figures ðŸ§
Want to see more data insights? Our free reports are just the right thing for you!