What is Categorical Data? Definition, Types, Examples
Appinio Research · 17.11.2023 · 29min read
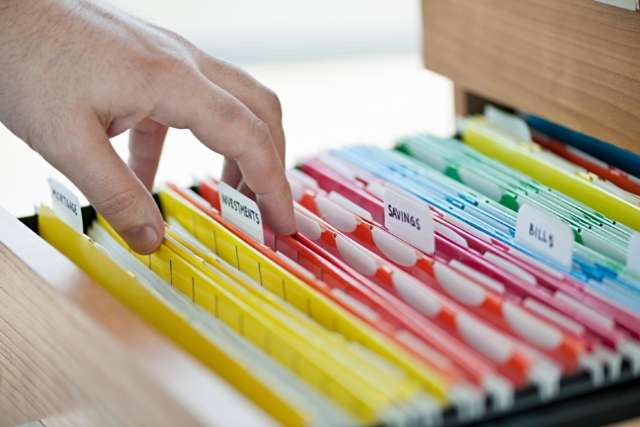
Content
Have you ever wondered how we make sense of the diverse categories that define our world? Categorical data, from gender to product types, are integral to understanding everything from consumer behavior to healthcare outcomes.
In this guide, we'll unravel the complexities of categorical data analysis, equipping you with the knowledge and techniques to decode the stories hidden within these distinct groups.
What is Categorical Data?
Categorical data, also known as qualitative data, is a type of data that represents discrete, distinct categories or groups. Unlike numerical data, which can be measured and quantified, categorical data fall into specific, non-numeric categories. Categorical data are often used to classify items or observations into different groups based on particular characteristics or attributes.
Categorical data can be further divided into two main types:
- Nominal Data: Categories have no inherent order or ranking in nominal data. Each category is distinct and unrelated to the others. Nominal data are often used for classification purposes.
Examples include gender (male, female, other), colors (red, blue, green), and countries (USA, Canada, UK). - Ordinal Data: Ordinal data, on the other hand, represent categories with a meaningful order or ranking, but the intervals between categories may not be uniform or precisely defined. Ordinal data allow you to express preferences or degrees of difference between categories.
Examples include education levels (high school, bachelor's degree, master's degree) and customer satisfaction ratings (very dissatisfied, dissatisfied, neutral, satisfied, very satisfied).
Categorical data are prevalent in various fields, such as market research, social sciences, healthcare, and more. Understanding the nature of categorical data is essential for conducting effective data analysis and drawing meaningful insights.
Importance of Categorical Data Analysis
Categorical data analysis is of paramount importance in the world of statistics and data science for several reasons:
- Decision Making: Categorical data analysis provides insights that help make informed decisions. For example, understanding customer preferences or voting patterns can guide marketing strategies and political campaigns.
- Pattern Recognition: Analyzing categorical data helps identify patterns and relationships within the data. This can lead to the discovery of valuable insights, such as consumer behavior trends or the impact of interventions in healthcare.
- Classification: Categorical data are often used for classification tasks, such as determining the product type, classifying emails as spam or not, or categorizing patient diagnoses.
- Hypothesis Testing: Categorical data analysis is fundamental for hypothesis testing to determine whether there is a statistically significant association between two categorical variables. This is commonly done using tests like the Chi-Square Test or Fisher's Exact Test.
- Predictive Modeling: Categorical data are frequently used in predictive modeling, where the goal is to predict outcomes or group memberships based on categorical predictors. Logistic regression is a common technique for this purpose.
- Policy and Strategy Formulation: Government policies, business strategies, and marketing campaigns often rely on categorical data analysis to understand public opinions, customer preferences, and market segmentation.
- Research and Academia: In academic research, categorical data analysis is employed to test hypotheses, validate theories, and contribute to the body of knowledge in various disciplines.
Categorical data analysis is vital in gaining insights, making informed decisions, and contributing to advancements in various fields. It enables researchers, analysts, and organizations to harness the power of qualitative data for a wide range of applications and purposes.
Types of Categorical Data
Categorical data can be classified into two main types: nominal data and ordinal data. Understanding the distinctions between these types is essential for practical data analysis and interpretation.
Nominal Data
Nominal data represents categories or groups with no inherent order or ranking. In other words, the categories are distinct and unrelated to each other. Nominal data is primarily used for classification purposes. Here are some examples:
- Color: Categories like "Red," "Blue," and "Green" have no inherent order.
- Gender: Categories like "Male," "Female," and "Other" are distinct, with no ranking.
- Country: Categories like "USA," "Canada," and "UK" represent distinct nations.
When working with nominal data, it's important to note that mathematical operations such as addition or subtraction are not meaningful because there is no inherent order among the categories. However, you can perform certain operations like counting the frequency of each category or calculating proportions.
Ordinal Data
Ordinal data, on the other hand, represents categories with a meaningful order or ranking, but the intervals between categories may not be uniform or precisely defined. This type of data allows you to express preferences or degrees of difference between categories. Here are some examples:
- Education Level: Categories like "High School," "Bachelor's Degree," and "Master's Degree" have a precise order, indicating increasing levels of education. However, the difference in knowledge between these categories may not be uniform.
- Customer Satisfaction: Categories like "Very Dissatisfied," "Dissatisfied," "Neutral," "Satisfied," and "Very Satisfied" reflect a degree of satisfaction. While there's a clear ranking, the intervals between these categories may not be equal.
Ordinal data analysis considers the order of categories but doesn't assume that the differences between them are consistent. This data type is common in surveys and questionnaires where respondents provide subjective assessments or rankings.
When working with ordinal data, it's essential to choose appropriate statistical methods that account for the ordinal nature of the data. Analyzing ordinal data using techniques designed for continuous data can lead to incorrect conclusions.
Understanding whether your data is nominal or ordinal is fundamental to selecting the proper analysis techniques and drawing meaningful conclusions from your categorical data. Both types offer valuable insights when handled correctly, and choosing the appropriate approach depends on the nature of your data and research questions.
How to Prepare Data for Categorical Data Analysis?
Proper data preparation is a crucial step in categorical data analysis, as it sets the foundation for accurate and insightful results. We'll explore the key aspects of preparing your categorical data for analysis.
1. Data Collection
Data collection involves the process of gathering categorical data from various sources, such as surveys, questionnaires, databases, or observations. The quality and accuracy of your data collection methods significantly impact the validity of your analysis. Here are some essential considerations for data collection:
- Clear Definitions: Ensure that categories are well-defined and mutually exclusive. Ambiguity in category definitions can lead to misclassification and unreliable results.
- Sampling Techniques: If your data is obtained through sampling, use appropriate techniques to ensure your sample is representative of the population you intend to study.
- Consistency: Maintain consistency in data collection methods over time to facilitate comparisons and trend analysis.
Effective data collection is the cornerstone of successful categorical data analysis. When it comes to streamlining your data collection processes, Appinio offers a user-friendly solution that simplifies the gathering of insights from a diverse audience.
With Appinio, you can efficiently collect categorical data through surveys and questionnaires, enabling you to focus on the analysis and interpretation of your findings.
Book a demo today to explore how Appinio can enhance your data collection efforts and lead to more informed decisions!
2. Data Encoding
Data encoding is the process of representing categorical data in a numerical format suitable for analysis. Since many statistical methods require numerical input, encoding is necessary to work with categorical data effectively. Common techniques include:
- One-Hot Encoding: For nominal data, you can use one-hot encoding, where each category is represented as a binary variable (0 or 1). Each category gets its column, and a 1 indicates the presence of that category.
- Ordinal Encoding: For ordinal data, assign numerical values to categories based on their order. Be cautious when assigning values, as they should reflect the inherent order of the categories without implying equal intervals between them.
Choosing the appropriate encoding method depends on the type of categorical data you're working with and the analysis you intend to perform. Understanding the implications of encoding choices on your analysis results is essential.
3. Handling Missing Values
Dealing with missing values, including categorical data, is a common challenge in data analysis. Missing values can introduce bias and affect the reliability of your results. Here's how to handle missing values in categorical data:
- Omitting Missing Data: You can choose to remove rows or cases with missing categorical values if they represent a small portion of your dataset, and their exclusion won't significantly impact your analysis.
- Imputation: Imputation involves replacing missing values with estimated or imputed values. For nominal data, you might replace missing values with the mode (the most frequent category). For ordinal data, you could use the median or a suitable imputation method.
Documenting how missing values were handled in your analysis is crucial, as this transparency ensures the reproducibility and trustworthiness of your findings.
4. Data Visualization for Categorical Data
Data visualization is a powerful tool for gaining insights into categorical data before conducting formal analysis. Visual representations of categorical data help you understand its distribution, relationships, and patterns. Here are some visualization techniques:
- Bar Charts: Bar charts are effective for visualizing the frequency of each category within a variable. They display categories on the x-axis and their frequencies or proportions on the y-axis.
- Pie Charts: Pie charts show the proportional distribution of categories within a variable, making it easy to see the relative sizes of each category.
- Stacked Bar Charts: Stacked bar charts allow you to simultaneously compare the composition of multiple categorical variables by stacking bars for different categories within each variable.
- Fishbone Diagram: A fishbone diagram is a visual tool used for root cause analysis. It helps identify and organize potential causes of a problem or an outcome by categorizing them into different branches, resembling the shape of a fishbone.
Data visualization provides valuable insights, such as identifying dominant categories, spotting outliers, and detecting potential data anomalies. It helps you make informed decisions about data encoding, analysis techniques, and research directions.
Thorough data preparation for categorical data analysis involves careful data collection, appropriate encoding methods, thoughtful handling of missing values, and insightful data visualization. These steps lay the groundwork for meaningful and accurate analysis, enabling you to draw valuable insights from your categorical data.
Descriptive Statistics for Categorical Data
Descriptive statistics are essential for gaining a clear understanding of categorical data. We'll explore various tools and techniques for effectively summarizing and visualizing categorical data.
Frequency Tables
Frequency tables, also known as contingency tables, are fundamental for summarizing categorical data. They display the count or percentage of observations within each category of a categorical variable. To create a frequency table:
- Identify the Categorical Variable: Determine which variable you want to summarize, and make sure it is categorical in nature.
- List Categories: List all unique categories within the variable. Ensure that you capture all possible categories.
- Count Observations: For each category, count the number of observations or occurrences in your dataset.
- Calculate Percentages: Optionally, you can calculate the percentage of observations in each category by dividing the count for each category by the total number of observations and multiplying by 100.
Frequency tables provide a concise summary of the distribution of categorical data, allowing you to identify which categories are most common or rare. They are especially useful when comparing different groups or variables.
Bar Charts
Bar charts are visual representations of categorical data that make it easy to compare the frequencies or proportions of different categories within a variable. To create a bar chart for categorical data:
- Select the Categorical Variable: Choose the categorical variable you want to visualize.
- Count Observations: Count the number of observations or occurrences within each category of the chosen variable.
- Create the Chart: Plot the categories on the x-axis and the corresponding frequencies or proportions on the y-axis. You can use vertical or horizontal bars.
Bar charts are effective for visualizing the distribution of categorical data and identifying dominant or significant categories. They are widely used in reports and presentations to communicate categorical data in a visually engaging way.
Pie Charts
Pie charts are another visualization tool for categorical data, illustrating the proportional distribution of categories within a variable. To create a pie chart for categorical data:
- Identify the Categorical Variable: Choose the categorical variable you want to represent in a pie chart.
- Count Observations: Count the number of observations or occurrences within each category of the chosen variable.
- Calculate Proportions: Calculate the proportion of each category by dividing its count by the total number of observations.
- Create the Chart: Represent each category as a slice of the pie, with the size of the slice proportional to the category's proportion.
Pie charts provide a visual overview of how categories contribute to the whole, making it easy to see the relative distribution of data. However, they are best suited for situations where the number of categories is small, as pie charts can become complex and challenging to interpret with many categories.
Descriptive statistics for categorical data, including frequency tables, bar charts, and pie charts, are valuable tools for summarizing and visualizing the distribution of categorical variables. These techniques help you uncover patterns, identify significant categories, and communicate your findings effectively to others.
Inferential Statistics for Categorical Data
Inferential statistics are a crucial part of categorical data analysis, allowing you to draw conclusions and make inferences about populations based on sample data. Let's delve into three essential inferential statistical techniques for categorical data: the Chi-Square Test, Fisher's Exact Test, and Odds Ratios.
Chi-Square Test
The Chi-Square Test is a statistical test used to assess the association or independence between two categorical variables. It is advantageous when you want to determine if there is a significant relationship between two categorical variables in a contingency table.
Here's how the Chi-Square Test works:
- Formulate Hypotheses: You start by formulating null (H0) and alternative (Ha) hypotheses. The null hypothesis assumes that there is no association between the variables, while the alternative hypothesis suggests that there is a significant association.
- Create a Contingency Table: Construct a contingency table that cross-tabulates the two categorical variables, showing the counts or frequencies of observations in each combination of categories.
- Calculate Expected Frequencies: Calculate the expected frequencies for each cell in the contingency table under the assumption of independence between the variables.
Compute the Chi-Square Statistic: Calculate the Chi-Square statistic, which measures the difference between the observed and expected frequencies. - Determine Significance: Compare the calculated Chi-Square statistic to the Chi-Square distribution to determine if the observed association is statistically significant.
The Chi-Square Test is widely used in various fields, including social sciences, healthcare, and market research, to analyze the relationships between categorical variables and make informed decisions.
Fisher's Exact Test
Fisher's Exact Test is a statistical test used to assess the association between two categorical variables when dealing with small sample sizes or rare events. It is valuable when the Chi-Square Test assumptions are not met.
Here's how Fisher's Exact Test works:
- Formulate Hypotheses: As with the Chi-Square Test, you start by formulating null (H0) and alternative (Ha) hypotheses regarding the association between the two categorical variables.
- Create a Contingency Table: Construct a contingency table that cross-tabulates the two categorical variables, showing the counts or frequencies of observations in each combination of categories.
- Calculate the Probability of the Observed Table: Using combinatorial mathematics, compute the probability of observing the given contingency table and all tables more extreme than the observed table under the assumption of independence.
- Determine Significance: Compare the calculated probability to a predetermined significance level (alpha) to determine if the observed association is statistically significant.
Fisher's Exact Test is valuable in situations where the Chi-Square Test may yield unreliable results due to small sample sizes or when dealing with 2x2 contingency tables. It is commonly used in medical research, genetics, and rare event analysis.
Odds Ratios
Odds Ratios (OR) are a measure of the strength and direction of the association between two categorical variables, often used in logistic regression analysis. The odds ratio quantifies the likelihood of an event occurring in one group compared to another group.
Here's how you calculate and interpret odds ratios:
- Calculating Odds Ratios: To calculate the odds ratio for a specific category of one variable compared to another, you take the ratio of the odds of the event occurring in one category to the odds of the event occurring in the reference category.
- Interpreting Odds Ratios: An odds ratio greater than 1 indicates that the event is more likely to occur in the first category compared to the reference category, while an odds ratio less than 1 suggests that the event is less likely.
Odds ratios are widely used in epidemiology, clinical studies, and predictive modeling to assess the impact of categorical variables on the likelihood of an outcome or event.
Inferential statistics for categorical data, including the Chi-Square Test, Fisher's Exact Test, and Odds Ratios, are powerful tools for analyzing relationships, associations, and the predictive strength of categorical variables. These methods help you make informed decisions, identify significant patterns, and draw meaningful conclusions from your categorical data analysis.
How to Model Categorical Data?
Modeling categorical data is a critical step in statistical analysis, allowing you to predict outcomes, assess associations, and gain deeper insights into the relationships between categorical variables. In this section, we'll explore three important modeling techniques for categorical data: Logistic Regression, Multinomial Logistic Regression, and Poisson Regression for Count Data.
Logistic Regression
Logistic Regression is a powerful statistical method used when the dependent variable is binary or categorical. It is helpful when you want to predict the probability of an event occurring based on one or more independent categorical or numeric variables.
- Model Formulation: Formulate the logistic regression model by specifying the dependent variable (binary or categorical) and one or more predictor variables. The model estimates the log-odds (logit) of the dependent variable being in a particular category.
- Parameter Estimation: Use statistical software to estimate the model parameters (coefficients) that maximize the likelihood of the observed data.
- Interpretation: Interpret the coefficients to understand the effect of each predictor variable on the log-odds of the outcome. Exponentiating the coefficients provides odds ratios, indicating the impact on the odds of the outcome.
- Prediction: Use the model to predict the probability of the dependent variable belonging to a specific category for new observations.
Multinomial Logistic Regression
Multinomial Logistic Regression extends logistic regression to situations where the dependent variable has more than two categories (i.e., it is multinomial). It is suitable for modeling categorical outcomes with three or more mutually exclusive categories.
- Model Formulation: Formulate the multinomial logistic regression model with the dependent variable having multiple categories. The model estimates log-odds for each category compared to a reference category.
- Parameter Estimation: Estimate the model parameters (coefficients) using appropriate software. The model assigns a set of coefficients to each predictor variable for each category.
- Interpretation: Interpret the coefficients for each category, considering them in relation to the reference category. Exponentiating the coefficients provides odds ratios for each category compared to the reference category.
- Prediction: Use the model to predict the probabilities of each category for new observations.
Multinomial logistic regression is useful in various fields, including political science (predicting voting behavior), marketing (brand choice analysis), and biology (species classification).
Poisson Regression for Count Data
Poisson Regression is a modeling technique designed for count data, which are discrete variables that represent the number of occurrences of an event within a fixed unit of time or space. It is instrumental when analyzing data with overdispersion or when the count data does not follow a normal distribution.
- Model Formulation: Formulate the Poisson regression model with a count-dependent variable and one or more predictor variables. The model estimates the expected count of events based on the predictors.
- Parameter Estimation: Estimate the model parameters (coefficients) using statistical software. The coefficients represent the effect of each predictor variable on the expected count.
- Interpretation: Interpret the coefficients to understand how each predictor variable impacts the expected count of events. Exponentiating the coefficients provides incidence rate ratios (IRRs), which quantify the multiplicative effect on the expected count.
- Prediction: Use the model to predict the expected events count for new observations.
Modeling categorical data using techniques like Logistic Regression, Multinomial Logistic Regression, and Poisson Regression is crucial for predicting outcomes, understanding relationships, and drawing meaningful insights from categorical variables with various levels of complexity. These models empower researchers and analysts across diverse fields to make data-driven decisions and explore the associations within their data.
How to Interpret and Report Categorical Data Analysis Results?
Interpreting and reporting the results of your categorical data analysis is a critical aspect of the research process. In this section, we'll explore how to effectively present your findings, discuss practical applications of categorical data analysis, and highlight common pitfalls and challenges to be aware of.
Presenting Results
Presenting your categorical data analysis results in a clear and concise manner is essential for conveying your findings to both technical and non-technical audiences.
- Tables and Charts: Use tables, bar charts, pie charts, and other graphical representations to visualize the distribution of categorical variables and any significant associations.
- Statistical Significance: Clearly indicate which results are statistically significant and which are not. Use appropriate symbols or annotations to denote significance levels.
- Descriptive Statistics: Provide descriptive statistics such as frequencies, percentages, and measures of central tendency for key categories.
- Effect Sizes: When applicable, include effect size measures like odds ratios or relative risk to convey the practical significance of relationships.
- Contextual Information: Offer context and explanations for observed patterns, associations, or trends. Discuss the implications of your findings and relate them to your research objectives.
- Limitations: Acknowledge any limitations of your analysis, such as sample size, data quality, or potential sources of bias.
- Visual Clarity: Ensure that your visuals are clear, properly labeled, and easy to interpret. Avoid clutter and excessive detail.
Practical Applications
Categorical data analysis has a wide range of practical applications across various fields. Understanding these applications can help you apply your analysis effectively.
Some practical areas where categorical data analysis is valuable include:
- Marketing and Market Research: Analyze customer preferences, buying behavior, and demographic segmentation to tailor marketing strategies.
- Healthcare: Study disease prevalence, treatment outcomes, and patient satisfaction to improve healthcare delivery.
- Social Sciences: Examine survey responses, voting patterns, and social behaviors to better understand human society.
- Finance and Insurance: Predict credit risk, insurance claims, and investment choices based on categorical variables.
- Epidemiology: Investigate disease outbreaks, risk factors, and public health interventions.
- Environmental Studies: Analyze species distribution, habitat preferences, and conservation efforts.
- Education: Assess student performance, teacher effectiveness, and educational outcomes.
Common Pitfalls and Challenges
Categorical data analysis, like any statistical analysis, comes with its share of challenges and potential pitfalls. Common issues to be aware of include:
- Small Sample Sizes: Small samples can lead to unreliable results, especially in Chi-Square and Fisher's Exact Tests. Consider the statistical power of your analysis.
- Bias and Nonresponse: Biased data collection methods or nonresponse can introduce selection bias, affecting the representativeness of your results.
- Misclassification: Inaccurate categorization of data can lead to incorrect conclusions. Ensure clear and consistent category definitions.
- Overfitting: In modeling, be cautious of overfitting, where a model fits the training data too closely and fails to generalize well to new data.
- Multicollinearity: In regression models, multicollinearity (high correlations between predictor variables) can make it challenging to identify the individual effects of variables.
- Interpretation Errors: Misinterpreting odds ratios, p-values, or significance levels can lead to incorrect conclusions.
- Data Reporting: Be transparent in reporting your data, including any missing values, outliers, or transformations applied.
Awareness of these pitfalls and challenges will help you conduct a more robust and reliable categorical data analysis and avoid drawing erroneous conclusions.
Effectively interpreting and reporting the results of categorical data analysis involves clear presentation, recognizing practical applications, and addressing common challenges. Properly conveying your findings allows you to make informed decisions, contribute to research and decision-making processes, and ultimately derive value from your categorical data analysis efforts.
Categorical Data Analysis Examples
To better understand how categorical data analysis works and its practical applications, let's explore a few real-world examples where categorical data plays a crucial role.
Market Segmentation
Imagine you work for a marketing agency tasked with creating targeted advertising campaigns for a new smartphone. To effectively reach potential customers, you need to perform market segmentation. Here's how categorical data analysis comes into play.
- Categorical Variables: In this scenario, categorical variables could include age groups (e.g., 18-24, 25-34, 35-44, etc.), income brackets (e.g., low-income, middle-income, high-income), and smartphone brand preferences (e.g., Apple, Samsung, Google, etc.).
- Analysis: By analyzing survey responses or customer data, you can use techniques like cross-tabulation, Chi-Square tests, or logistic regression to identify patterns. For instance, you may discover that the majority of 18-24-year-olds prefer smartphones from a particular brand, allowing you to tailor your marketing strategies accordingly.
- Application: This categorical data analysis helps you create targeted advertising campaigns that resonate with specific demographic groups, maximizing the effectiveness of your marketing efforts.
Medical Diagnosis
In the field of healthcare, categorical data analysis is instrumental in diagnosing and treating diseases. Consider a scenario involving a medical researcher studying the relationship between smoking habits and lung cancer.
- Categorical Variables: The researcher collects data on smoking habits, categorizing individuals as non-smokers, occasional smokers, and heavy smokers. The outcome variable is the presence or absence of lung cancer (categorized as "yes" or "no").
- Analysis: Using categorical data analysis techniques like Chi-Square tests or logistic regression, the researcher can assess whether there is a statistically significant association between smoking habits and lung cancer. The analysis may reveal that heavy smokers have a significantly higher risk of developing lung cancer compared to non-smokers.
- Application: This analysis provides valuable insights for healthcare professionals and policymakers, helping them develop targeted anti-smoking campaigns and preventive measures to reduce lung cancer rates.
Customer Feedback Analysis
Analyzing feedback and reviews in customer service is essential for improving products and services. Let's explore how categorical data analysis can be applied:
- Categorical Variables: Customer feedback is often categorized into sentiment categories such as "positive," "neutral," and "negative." Additionally, feedback can be further categorized by product features or aspects that customers mention.
- Analysis: Through sentiment analysis and cross-tabulation, you can identify trends in customer sentiment. For example, you may discover that customers consistently praise a specific feature but express dissatisfaction with another.
- Application: Armed with this analysis, businesses can prioritize improvements, allocate resources effectively, and enhance customer satisfaction by addressing pain points and improving positive aspects of their products or services.
These examples illustrate how categorical data analysis is not only valuable but also versatile in its applications. Whether you're targeting marketing efforts, making critical healthcare decisions, or improving customer experiences, a solid grasp of categorical data analysis empowers you to make data-driven choices that drive success and positive outcomes in various fields.
Conclusion for Categorical Data
We've navigated the fascinating realm of categorical data, unlocking its potential to uncover insights and shape decisions. From understanding the types of categorical data to mastering inferential statistics, modeling techniques, and effective reporting, you now possess the tools to harness the power of qualitative information.
Categorical data analysis empowers you to unravel patterns, make informed choices, and contribute to diverse fields like marketing, healthcare, and social sciences. Remember, clarity, context, and careful interpretation are your guiding stars as you embark on your data analysis endeavors. Now, with this newfound knowledge, you're ready to embark on your own analytical journey, armed with the ability to explore, explain, and enlighten with categorical data.
How to conduct Categorical Data Analysis in Minutes?
In a world where speed and precision are paramount, Appinio emerges as the ultimate solution for obtaining categorical data with unparalleled ease. As a real-time market research platform, Appinio empowers companies to harness the benefits of swift, data-driven decision-making through its innovative approach.
- Lightning-Fast Insights: Appinio delivers answers in mere minutes, eliminating the waiting game and enabling swift decision-making.
- User-Friendly Interface: No need for a Ph.D. in research – Appinio's intuitive platform allows anyone to collect and analyze categorical data effortlessly.
- Global Reach: With access to a diverse array of target groups across 90+ countries, you can survey your desired demographics with precision and accuracy.
Get facts and figures ðŸ§
Want to see more data insights? Our reports are just the right thing for you!